Future of AI and Open Source in Data Science: Insights from ...
AI and open source have emerged as essential tools for businesses seeking to enhance efficiency and drive innovation. The intersection of these two transformative forces has a significant impact on the data science community, offering new opportunities while also presenting challenges.

According to a recent report by Anaconda, an overwhelming majority (87%) of data science practitioners are spending more time or as much time on AI techniques compared to last year. These techniques include using generative adversarial networks (GANs), deep learning, and transformer models.
Despite the growing adoption of AI, some companies face challenges such as budget constraints and lack of support to drive business value. Additionally, there is a sense of unpreparedness among respondents in handling data science challenges like government regulations and the steep learning curve for technology tools.
It is interesting to note that fewer people fear AI will take their jobs, as they are integrating AI into their workflows to handle repetitive tasks, allowing them to focus on more innovative pursuits.
The top use cases of AI, as per the report, include data cleaning, visualization, analysis, automating tasks, and prediction or detection models.
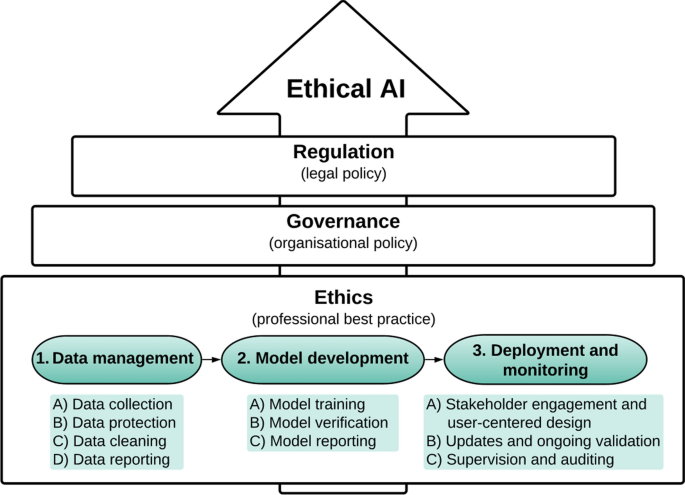
On the other hand, the key benefits of open-source software are speed of innovation, cost-effectiveness, and flexibility for developers to tailor solutions to specific project needs.
Challenges and Concerns
One of the significant challenges in AI adoption is open-source security, cited by 42% of respondents. The transparent and accessible nature of open-source code makes it vulnerable to security threats from malicious actors.
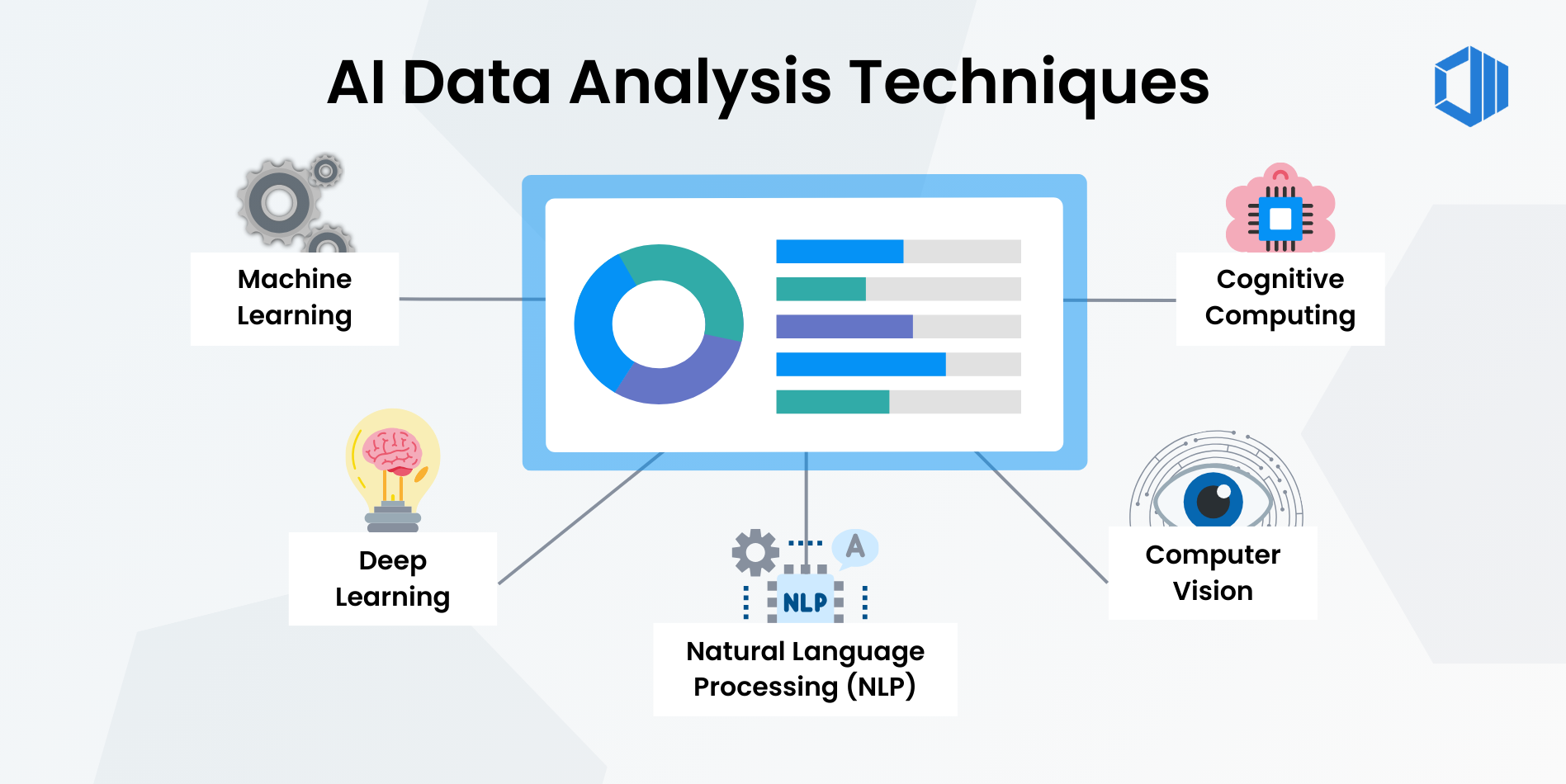
The 7th Annual Data Science Report by Anaconda, based on a survey of over 3000 professionals from 136 countries, sheds light on crucial concerns in the data science community. The need for regulations to ensure the explainability and transparency of AI models, addressing bias in algorithms, and promoting collaboration between academia and industry remain pressing issues.
Importance of Collaboration
Collaboration is emphasized as key to addressing these challenges by fostering open dialogue, learning, and problem-solving within the data science community. Organizations are urged to create environments that support collaborative ecosystems to drive innovation and overcome obstacles like security risks associated with AI and open-source tools.
Education and training play a vital role in nurturing the workforce through the early stages of the AI technological shift. Online courses, workshops, in-person training programs, peer learning, and mentorship are recommended methods for educating and upskilling data science practitioners and IT professionals.
Continuous learning, communication, and collaboration are identified as essential elements for maximizing the value derived from AI and open-source tools in data science.