Navigating fairness: practitioners' understanding, challenges, and strategies for AI/ML systems
The rise in the use of AI/ML applications across industries has raised concerns about the fairness of AI/ML systems. While previous research has touched on this topic, there is a lack of empirical studies focusing on the perspectives and experiences of AI practitioners in developing fair AI/ML systems. Understanding the views and experiences of AI practitioners is crucial as they play a direct role in the development and deployment of these systems, providing valuable real-world insights into the challenges of ensuring fairness.
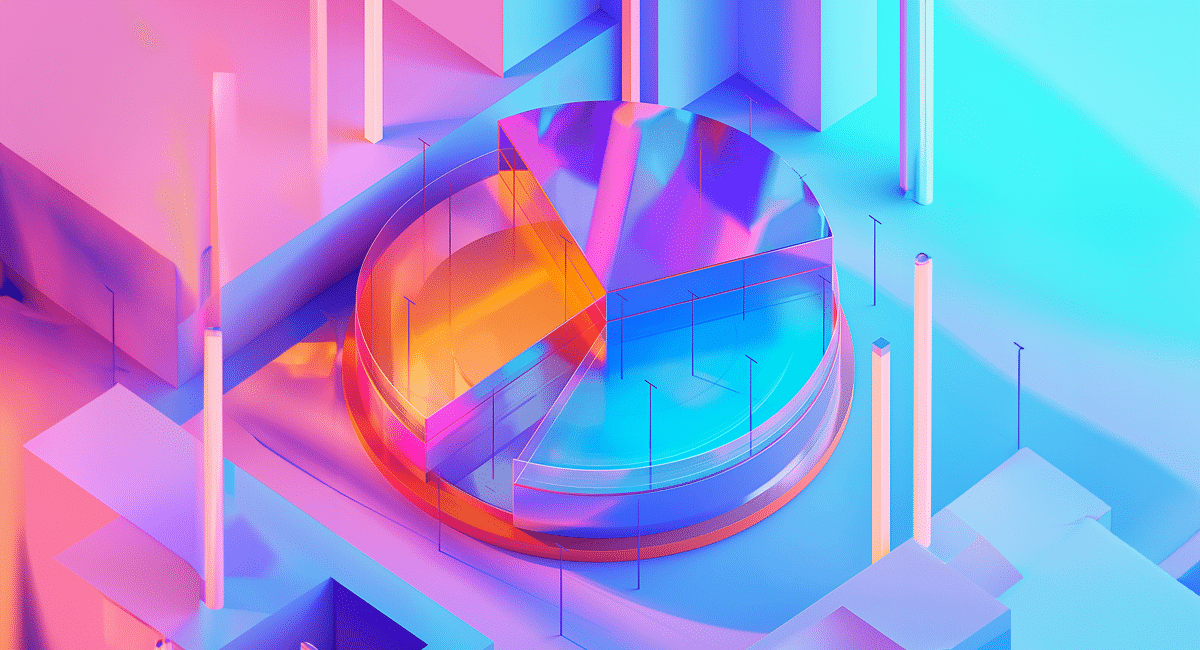
Insights from AI Practitioners
Through semi-structured interviews with 22 AI practitioners, we explored their understanding of 'fair AI/ML', the challenges they encounter in achieving fairness, the consequences of developing unfair systems, and the strategies they employ to promote fairness. By delving into the perspectives and experiences of AI practitioners, this study aims to offer actionable insights to enhance the fairness of AI/ML systems, reduce bias, and build public trust in AI technologies.
The Evolution of Fairness in AI/ML
The concept of 'fairness' in AI/ML systems has been a subject of interest in Software Engineering (SE) research for some time, preceding the current proliferation of AI/ML applications. Recent real-world incidents have underscored the importance of fairness in AI/ML systems. For instance, cases of gender bias in Google's ML algorithm and Amazon's recruitment tool have drawn attention to the repercussions of biased systems on users, particularly those from minority or disadvantaged groups.
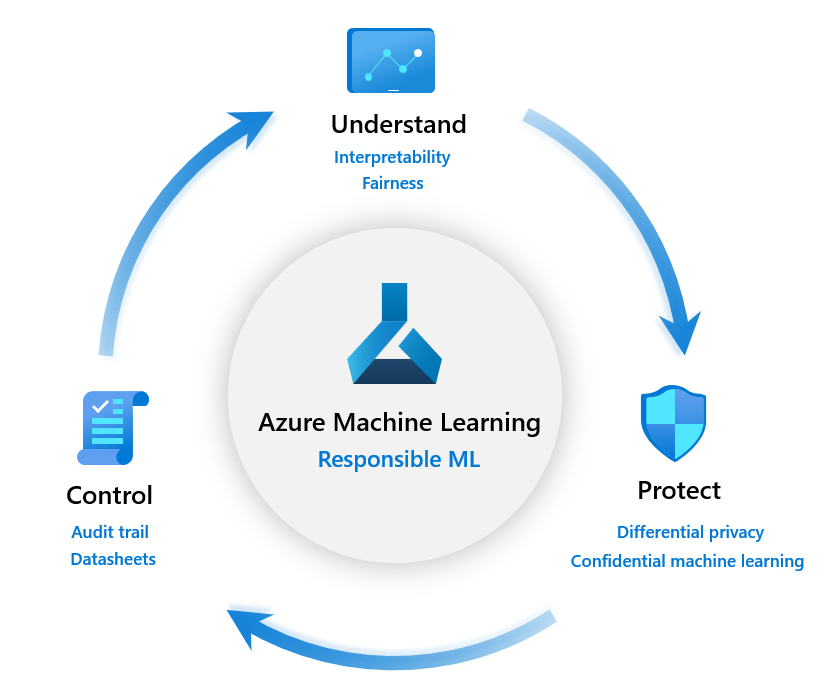
Prioritising Fairness in AI/ML Development
The widespread adoption of AI/ML systems in various domains has prompted increased scrutiny on fairness, leading to the development of guidelines and policies. Tech companies and regions like Australia and Europe have emphasized 'fairness' as a fundamental principle for AI practitioners in creating inclusive and non-discriminatory systems. To support this objective, tools and frameworks such as fairness toolkits, checklists, and FactSheets have been introduced to measure and promote fairness in AI/ML applications.
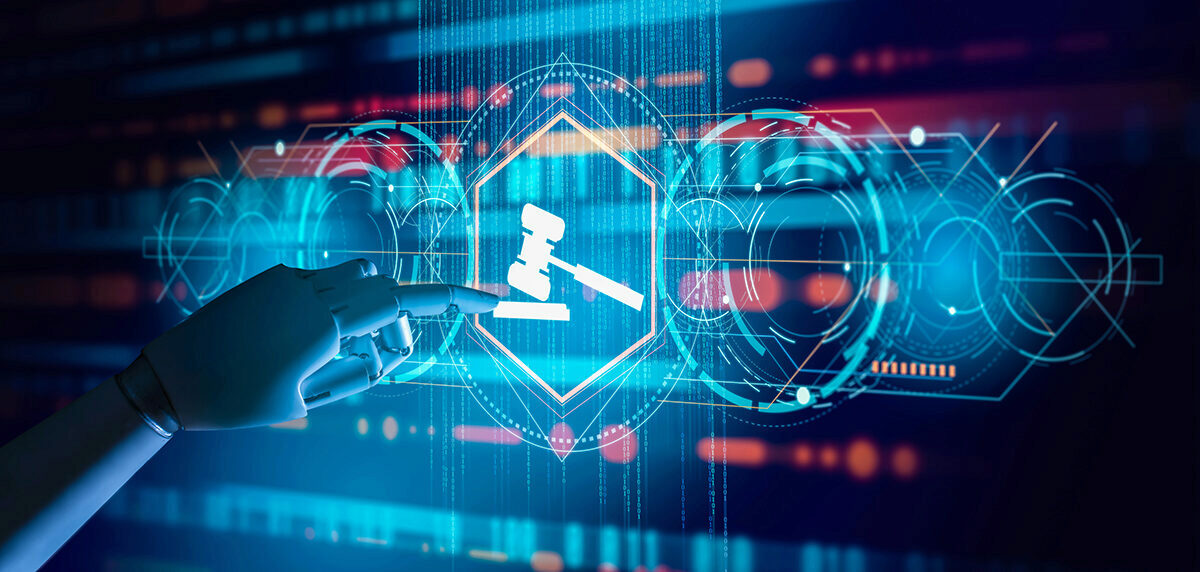
By prioritizing fairness in the development and deployment of AI/ML systems, practitioners can mitigate bias, promote inclusivity, and enhance the overall trustworthiness of AI technologies.