WashU Expert: How DeepSeek changes the AI industry - The Source
DeepSeek AI surged in popularity in the past week after analysts showed that it was trained much more efficiently compared with models like Open AI ChatGPT and other machine-learning platforms. According to a report by Ben Thompson, who provides tech industry analysis on his website Stratechery, DeepSeek was designed under constraints that ultimately led to innovation in how much computing power was required for the machine learning. The startup that developed DeepSeek is based in China, which is under a trade embargo that cuts access to high-quality semiconductor chips produced by Nvidia, an American multinational corporation.

Working with mostly lower-quality chips forced the DeepSeek developers to incorporate a variety of artificial intelligence (AI) optimization techniques that give their machine-learning platform vastly more bang for its buck. The DeepSeek developers claim it took roughly $5.6 million to train their latest version of the AI versus the most recent Chat GPT 4 training that reportedly cost $78 million and Google’s Gemini Ultra cost $191 million, according to Stanford University’s 2024 Artificial Intelligence Index report.
Impact on the AI Industry
Scientists at Washington University in St. Louis stand to benefit, as do consumers, when the costs of AI training are vastly reduced. Umar Iqbal, an assistant professor of computer science and engineering at the McKelvey School of Engineering, said his lab alone needs to spend tens of thousands of dollars to access these platforms and that competition from the Chinese startup will potentially lower prices.
One example of how DeepSeek reduced training costs, noted in the Stratechery post, is that their developers made use of a method called “distillation,” where they use an established generative AI system like ChatGPT to “teach” their system how to do the job. PhD students from McKelvey Engineering most recently tried distillation to improve large-language models without additional training.
Challenges and Concerns
For technologies to be mass adopted, they need to be cheap. What this has demonstrated is using models can become very cheap. Overall, this is an interesting development. It significantly reduced the cost of AI. We’ll be able to conduct experiments, more large-scale experiments. However, Iqbal warned of other pitfalls ahead.

To run these models, a person needs access to large-scale hardware; it’s not something somebody can download on their phone. The way AI platforms work is one person’s machine and data reaches out to the AI machine in the cloud — and that’s where they can potentially lose control of their data. “And that is a very serious concern,” Iqbal said.
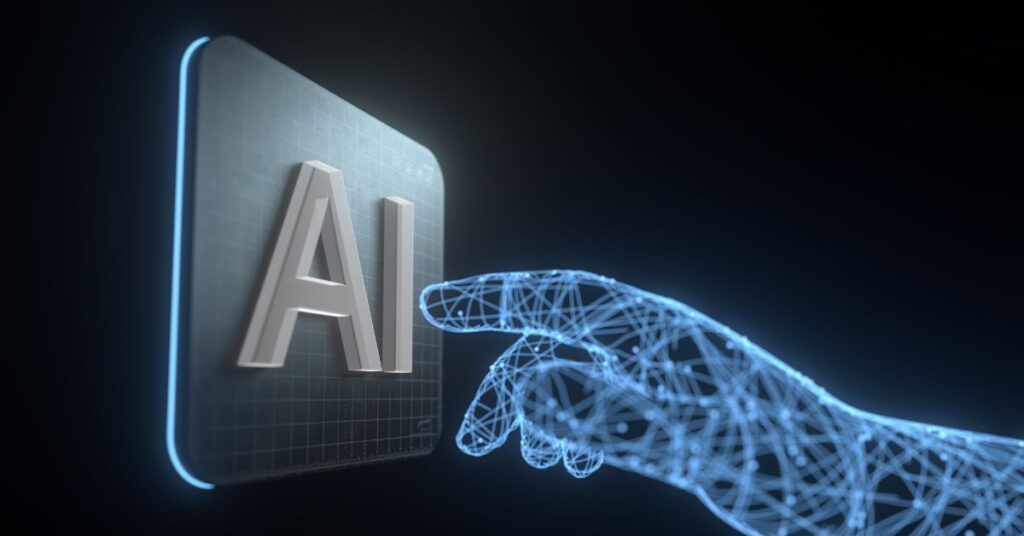
AI systems allow for vast surveillance infrastructure, some of which already is in place in the form of search engines that track user data from all over the web, mostly to further e-commerce. “All this data will go to different AI vendors, and they can use that information to profile users, infer their interests, surveil them and maybe also influence them,” Iqbal said.
Another concern is the increasing integration of AI language models into mobile apps. One increasingly advertised use for AI is in planning vacations. As the AI engages a variety of apps to do this, if any malicious software is lurking, it can potentially harvest more data from the user and manipulate the results the AI is seeking. “The technologies, when they have a lot of potential, they evolve very quickly,” Iqbal said. “You need to have guard rails and protections buried into the design. This is not happening with AI systems.”