The Exploratory Journey of AI and Web3 Convergence and Innovation
Web3, as a decentralized, open, and transparent new paradigm of the internet, has an inherent synergy with AI. Under the traditional centralized architecture, AI computing and data resources are subject to strict control, facing numerous challenges such as computational bottlenecks, privacy breaches, and algorithmic black boxes. On the other hand, Web3, built on distributed technologies, can infuse new vitality into AI development through shared computing networks, open data markets, and privacy-preserving computation. Simultaneously, AI can empower Web3 with capabilities like optimizing smart contracts and anti-cheating algorithms, aiding in the ecosystem's construction. Therefore, exploring the convergence of Web3 and AI is crucial for building the next-generation internet infrastructure and unlocking the value of data and computing power.
Data as the Core Driving Force
Data is the core driving force behind AI development, akin to fuel for an engine. AI models require ingesting vast amounts of high-quality data to gain profound understanding and robust reasoning abilities. Data not only provides the training foundation for machine learning models but also determines their accuracy and reliability.
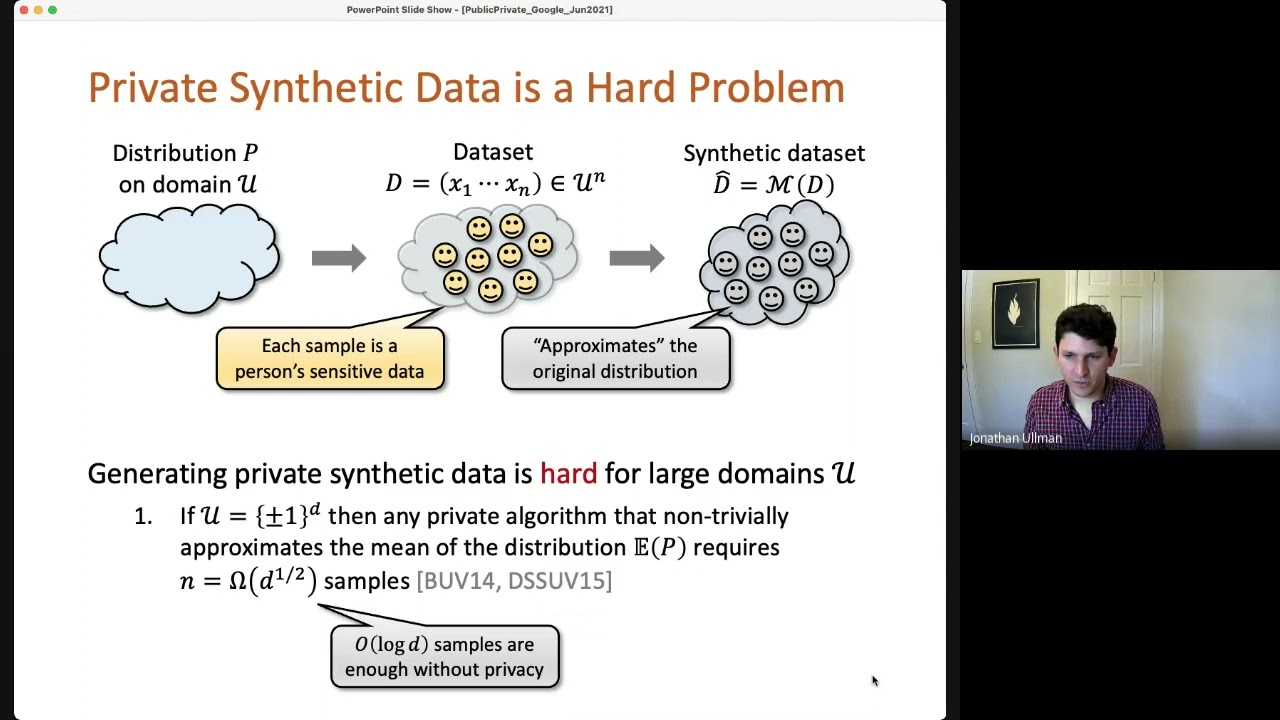
In the traditional centralized AI data acquisition and utilization model, several key issues arise:
Decentralized Data Paradigm of Web3
Web3 offers a new decentralized data paradigm to address the pain points of traditional models:
Nevertheless, real-world data acquisition also faces challenges such as varying data quality, high processing complexity, and insufficient diversity and representativeness. Synthetic data may be the rising star in the Web3 data realm. Based on generative AI technologies and simulations, synthetic data can mimic the properties of real data, effectively complementing it and improving data usage efficiency. In domains like autonomous driving, financial market trading, and game development, synthetic data has already demonstrated its mature application potential.
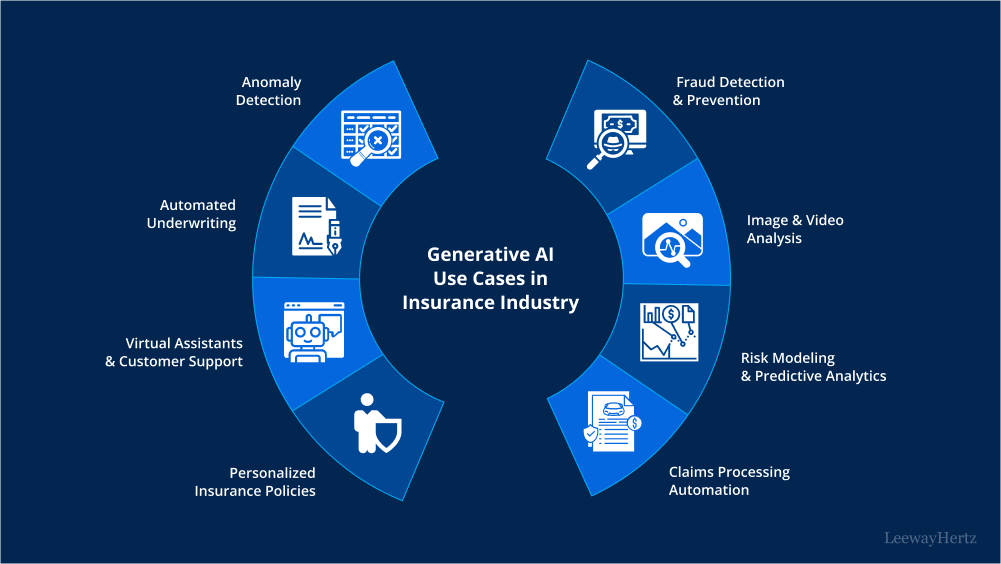
Privacy Protection and Fully Homomorphic Encryption (FHE)
FHE, or Fully Homomorphic Encryption, allows direct computation on encrypted data without the need for decryption, while the computation results are consistent with performing the same operations on plaintext data.
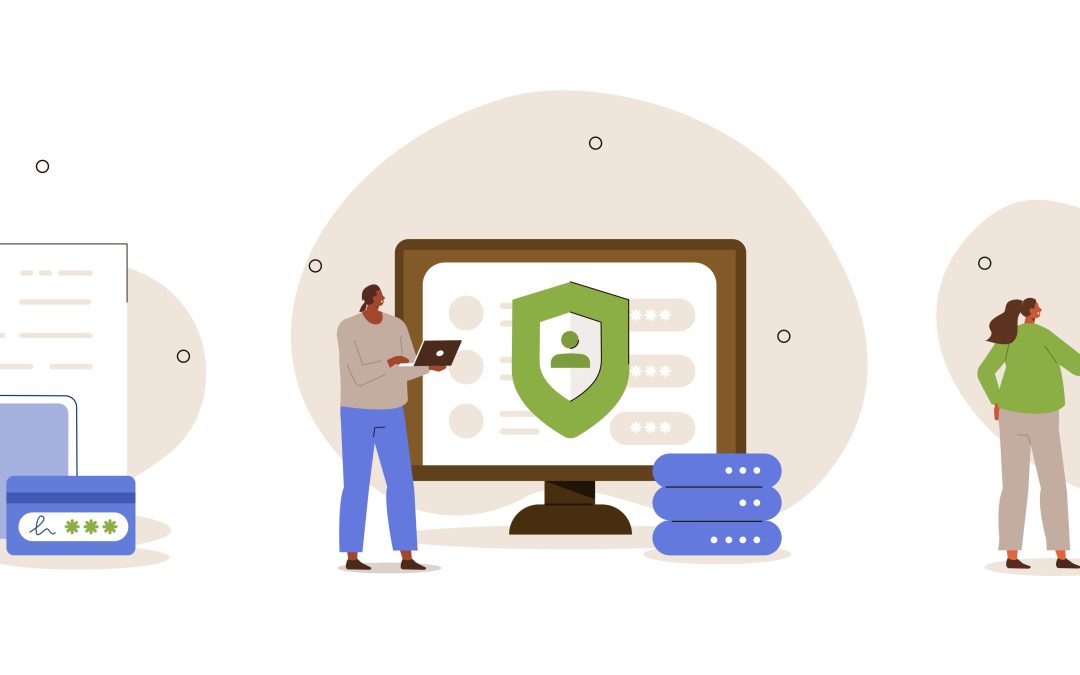
FHE provides robust protection for AI privacy computation, enabling GPU computing power to execute model training and inference tasks without accessing the original data. This presents significant advantages for AI companies, as they can securely open API services while protecting trade secrets.
Decentralized AI Computing Power Networks
The computational complexity of current AI systems doubles every three months, leading to a surge in computing power demands that far exceeds the supply of existing computing resources. IO.net is a decentralized AI computing power network based on Solana, aggregating idle GPU resources globally to provide AI companies with an economical and accessible computing power market.
In addition to general decentralized computing power networks, there are platforms dedicated to AI training, such as Gensyn and Flock.io, as well as specialized computing power networks focused on AI inference, like Ritual and Fetch.ai. Decentralized computing power networks provide a fair and transparent computing power market, breaking monopolies, lowering application barriers, and improving utilization efficiency.

Edge AI and DePIN in the Web3 Realm
Imagine your smartphone, smartwatch, or even smart home devices possessing the ability to run AI – this is the allure of Edge AI. It enables computation to occur at the data source, realizing low latency and real-time processing while protecting user privacy.
In the Web3 realm, DePIN enhances user privacy protection by processing data locally, reducing the risk of data leakages. Web3's native token economy can incentivize DePIN nodes to provide computing resources, building a sustainable ecosystem.
Initial Model Offering (IMO) and AI Agents
The concept of IMO (Initial Model Offering) tokenizes AI models, providing a novel method of funding and value-sharing for open-source AI models. Investors can purchase IMO tokens to share in the subsequent revenue generated by the model.
AI Agents can perceive their environment, engage in independent thinking, and take appropriate actions to achieve predefined goals. They can function as virtual assistants, learning user preferences through interactions and providing personalized solutions. Myshell is an open AI-native application platform offering a comprehensive and user-friendly toolset for configuring bot functionalities.
Future of Web3 and AI Convergence
In the convergence of Web3 and AI, the current focus is primarily on exploring the infrastructure layer, addressing critical issues such as acquiring high-quality data, protecting data privacy, hosting models on-chain, and improving the efficient utilization of decentralized computing power. As these infrastructural components gradually mature, we have reason to believe that the fusion of Web3 and AI will give birth to a series of innovative business models and services.