A synthetic solution for AI as chatbots run out of road | Mirage News
A leading UNSW computer scientist has highlighted that a potential solution for generative AI may not be the best fit for AI chatbots like ChatGPT and Google Gemini. These chatbots are facing a shortage of quality data, as most of the data they are legally allowed to process has been consumed, leading to concerns that by 2032, these chatbots may run out of good data.

Industry experts are now looking towards 'synthetic data' as a possible remedy for this data scarcity issue. Synthetic data refers to data that is either generated by AI based on real-world information or data that has been manipulated or edited by humans.
The Role of Synthetic Data in AI Development
ChatGPT creator, Sam Altman, believes that chatbots will eventually be able to train themselves solely on synthetic data. However, UNSW Computer Science Professor Claude Sammut raises doubts about this approach, highlighting the limitations of generative AI systems like chatbots.
Generative AI systems, such as ChatGPT, rely on pattern matching and struggle with logical sequential reasoning. While they can generate whole documents based on the texts they have seen before, they lack critical thinking abilities.
A recent study on 'model collapse' demonstrated the limitations of generative AI, where an image generator fed on its own output, resulting in a degraded image quality over multiple iterations.
The Role of Classical AI and Synthetic Data
'Classical AI', which represents knowledge in the form of symbols and rules, plays a crucial role in complementing generative AI systems. While synthetic data may not fully address the data shortage issue for generative AI, it has proven useful in applications like training robot soccer teams.
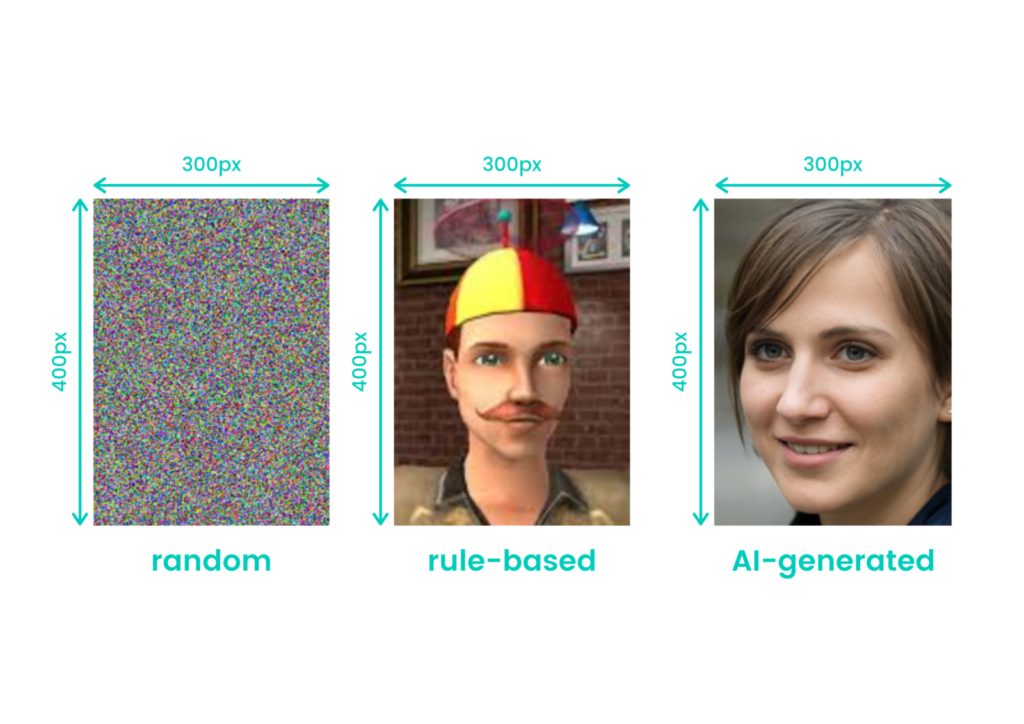
Prof. Sammut's rUNSWift team utilizes synthetic data to train its players by applying transformations to sample images. This approach has been successful, with the team winning five world titles since 2000.
Synthetic Data in Self-Driving Cars
The use of synthetic data extends beyond chatbots and robot soccer teams to industries like self-driving cars. A recent study comparing accident data between self-driving cars and human-driven cars revealed that self-driving cars are generally safer but face challenges during low-light conditions like sunrise and sunset.
Companies developing self-driving cars in the US are turning to synthetic data for training purposes. Despite the advancements, Prof. Sammut emphasizes that uncertainty will always be present in autonomous vehicles, as they strive to achieve reliability comparable to or better than human drivers.
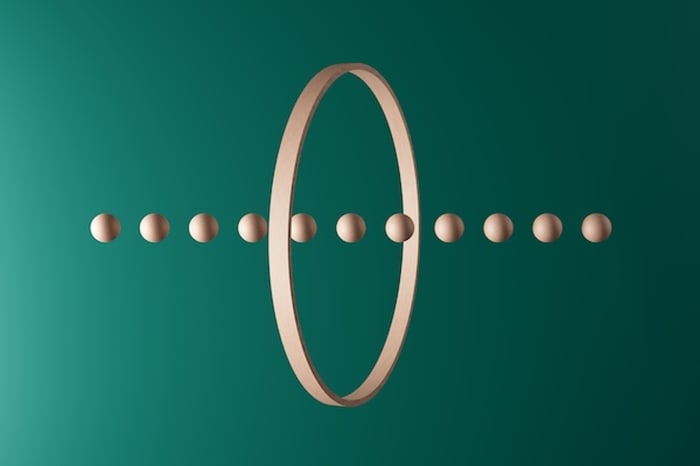
It is evident that synthetic data plays a pivotal role in addressing data shortages and enhancing the capabilities of AI systems across various industries, steering innovation and progress in the field of artificial intelligence.