Meta AI Introduces Collaborative Reasoner (Coral): An AI Breakthrough in Collaborative Reasoning
Large language models (LLMs) have shown impressive abilities in single-agent tasks like question answering and structured reasoning. However, the capability for collaborative reasoning, where multiple agents engage, disagree, and align on solutions, is still evolving.
The Need for Collaborative Reasoning
Collaborative reasoning is essential in various human tasks, from academic collaboration to decision-making in professional settings. Unfortunately, most LLM training procedures and benchmarks focus on individual, single-turn outputs, neglecting the social aspects of problem-solving like assertiveness, perspective-taking, and persuasion.
Introducing Collaborative Reasoner (Coral)
To address this gap, Meta AI has introduced Collaborative Reasoner (Coral), a framework tailored to assess and enhance collaborative reasoning skills in LLMs. Coral transforms traditional reasoning challenges into multi-agent, multi-turn tasks, where agents must not only solve problems but also come to a consensus through natural dialogue.
These interactions mirror real-world social dynamics, necessitating agents to challenge incorrect conclusions, negotiate conflicting viewpoints, and agree on collective decisions.
Framework Evaluation and Metrics
Coral spans five domains, including mathematics (MATH), STEM multiple-choice (MMLU-Pro, GPQA), and social cognition (ExploreToM, HiToM). These domains act as test environments to evaluate whether models can apply their reasoning skills in a cooperative, dialogue-driven context.
Coral introduces new evaluation metrics customized for multi-agent scenarios. At the conversation level, agreement correctness gauges whether agents converge on the correct solution. At the turn level, social behaviors like persuasiveness and assertiveness are explicitly quantified.
Data Generation and Training
To overcome the data challenge, Meta AI proposes a self-collaboration approach, where a single LLM plays both agent roles in a conversation. These synthetic dialogues are utilized to generate training data through a pipeline involving tree sampling, belief filtering, and preference fine-tuning using Direct Preference Optimization (DPO).

For efficient data generation, Meta introduces Matrix, a high-performance serving framework. Matrix supports various backends, employs gRPC for networking efficiency, and integrates with Slurm and Ray for large-scale orchestration.
Empirical Results and Future Implications
Evaluations across five benchmarks demonstrate that collaboration, when appropriately modeled and trained, yields significant improvements. Coral-trained models outperform baseline single-agent approaches in collaborative reasoning tasks.
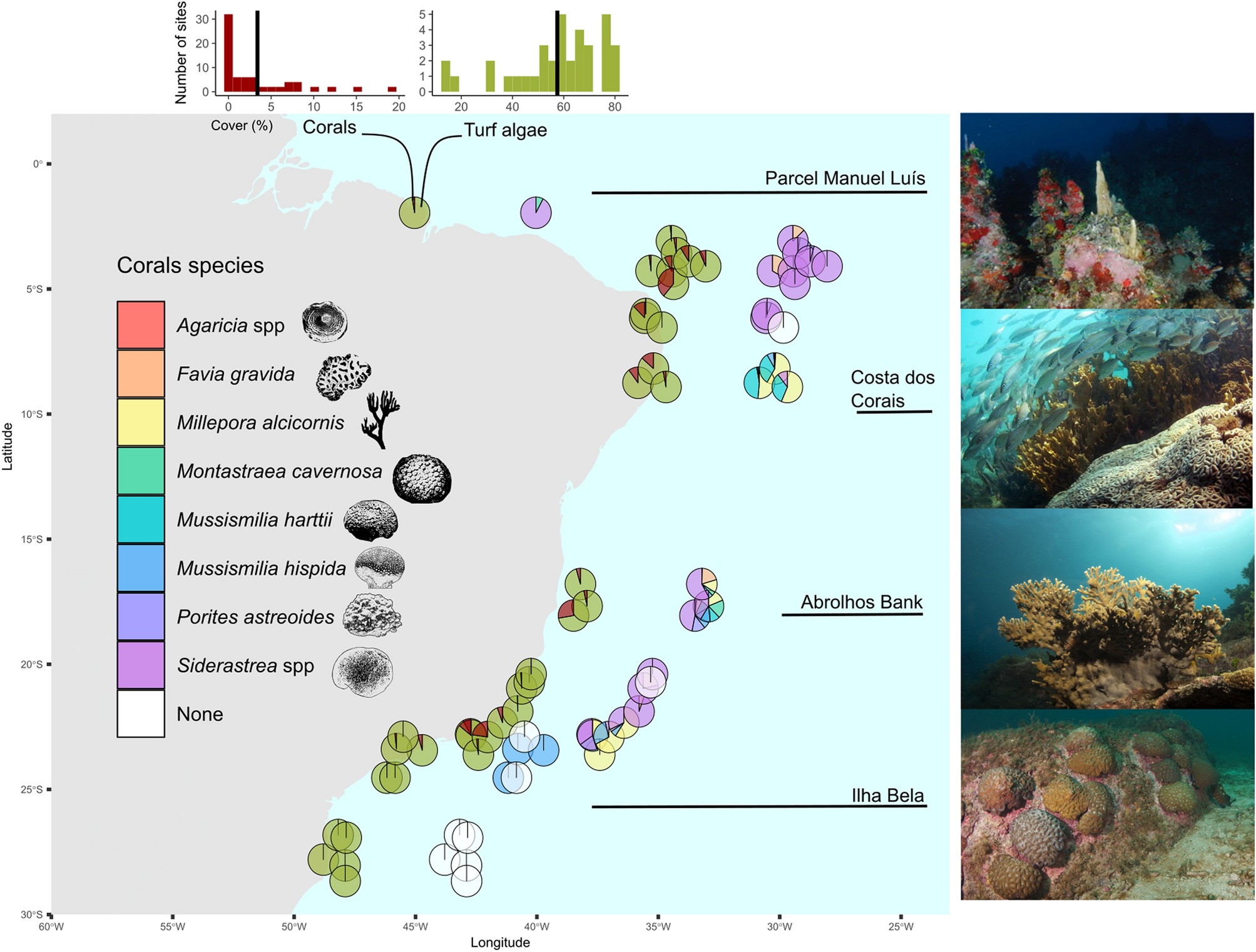
Despite these advancements, Coral-trained models still lag behind on complex mathematical problems, hinting that collaboration alone may not suffice in domains requiring deep symbolic reasoning.
Collaborative Reasoner establishes a structured and scalable pathway to evaluate and enhance multi-agent reasoning in language models. By combining synthetic self-dialogue and targeted social metrics, Meta AI introduces a unique approach to nurturing LLMs capable of effective collaboration.

As language models become more prevalent in human workflows, the ability to collaborate effectively is set to become a crucial skill. Coral represents a step in that direction, laying the groundwork for future research on social agents adept at navigating intricate, multi-agent environments.