When AI meets counterfactuals: the ethical implications of ...
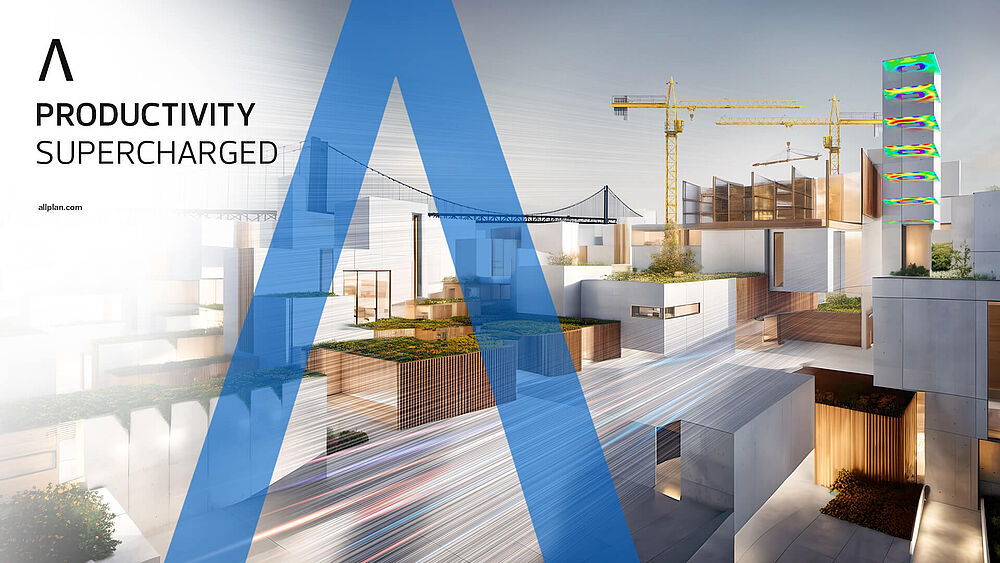
This paper explores the transformative potential of Artificial Intelligence (AI) integrated with counterfactual world simulation models (CWSMs). A CWSM leverages multimodal evidence, such as CCTV footage of a road accident, to create a detailed 3D reconstruction of the event. By simulating relevant counterfactual scenarios, it can address causal inquiries, such as determining if the accident occurred due to the driver's speeding. A normative and ethical framework is proposed to guide and regulate the simulation of counterfactuals, emphasizing the importance of maintaining fidelity in reconstructions while avoiding perpetuating stereotypes.
Interactions with AI-powered CWSMs
The paper anticipates various ways in which users will engage with AI-powered CWSMs and discusses the potential presentation of their outputs. It also delves into the possible applications of CWSMs in the legal field, acknowledging their capacity to transform legal proceedings while acknowledging the ethical dilemmas they raise. By outlining a new category of AI, this study aims to shed light on the responsible and efficient utilization of CWSMs.

AI-enabled Evidence Reconstruction
Utilizing AI, particularly multi-modal generative models, allows for the generation and analysis of evidence on a broader scale. These models can reconstruct past events based on diverse data sources, enabling the creation of realistic simulations. By integrating CCTV footage with world models of car dynamics and pedestrian behavior, AI-powered simulation models can generate 3D simulations of accidents and even conduct counterfactual simulations.
Advancements in Generative AI
The development of dynamic and precise 3D environments has long been a goal in generative AI. Modern Multimodal Generative Models can process multi-modal inputs and engage in counterfactual reasoning, expanding the possibilities for content generation and comprehension. The term "counterfactual world simulation models" (CWSMs) encompasses AI models that integrate various modalities into a world model to carry out counterfactual reasoning.
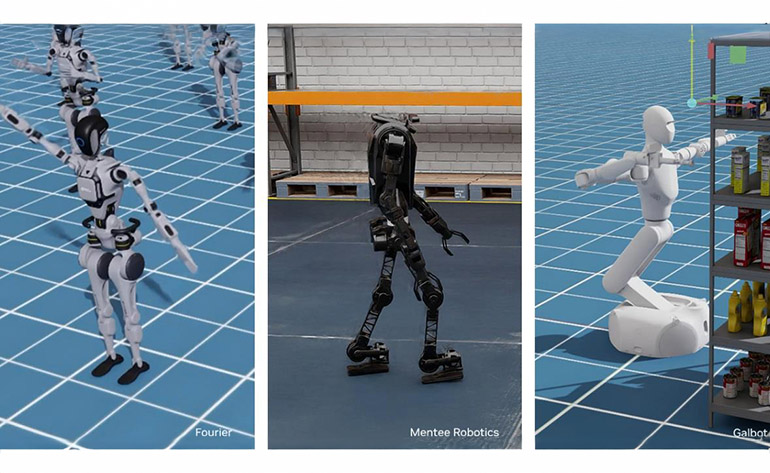
Evolution of CWSMs
CWSMs mark a shift from traditional image-generating AI by creating digital replicas of real-world scenarios utilizing different forms of evidence. These models depict the dynamic interactions of human agents within a physical environment over a specific timeframe, offering a new perspective on causality and responsibility.