AI's Fairness Problem: When Treating Everyone the Same is the ...
Current generative AI models are facing challenges in recognizing when demographic distinctions are significant, resulting in inaccurate, misleading, and sometimes harmful outcomes. For example, Google Gemini, when asked to produce images of Founding Fathers, depicted individuals from different demographic backgrounds. Similarly, when asked to generate images of Nazis, it produced historically inaccurate results, sparking backlash and controversy from various individuals and communities.
The Core Issue
The problem extends beyond image generation and calls into question the development, training, and alignment of generative AI models, especially large language models (LLMs). The prevailing notion of fairness in generative AI often relies on a flawed premise of complete blindness to demographic considerations. While the intention may be to promote equality, this approach can have unintended consequences and lead to biased outcomes.
Reimagining Fairness
In a recent paper published on the preprint server arXiv, a concept of "difference awareness" is introduced. Difference awareness refers to a model's ability to treat groups differently when necessary, emphasizing the importance of contextual understanding. This approach recognizes that fairness does not always require treating all groups in the same manner.
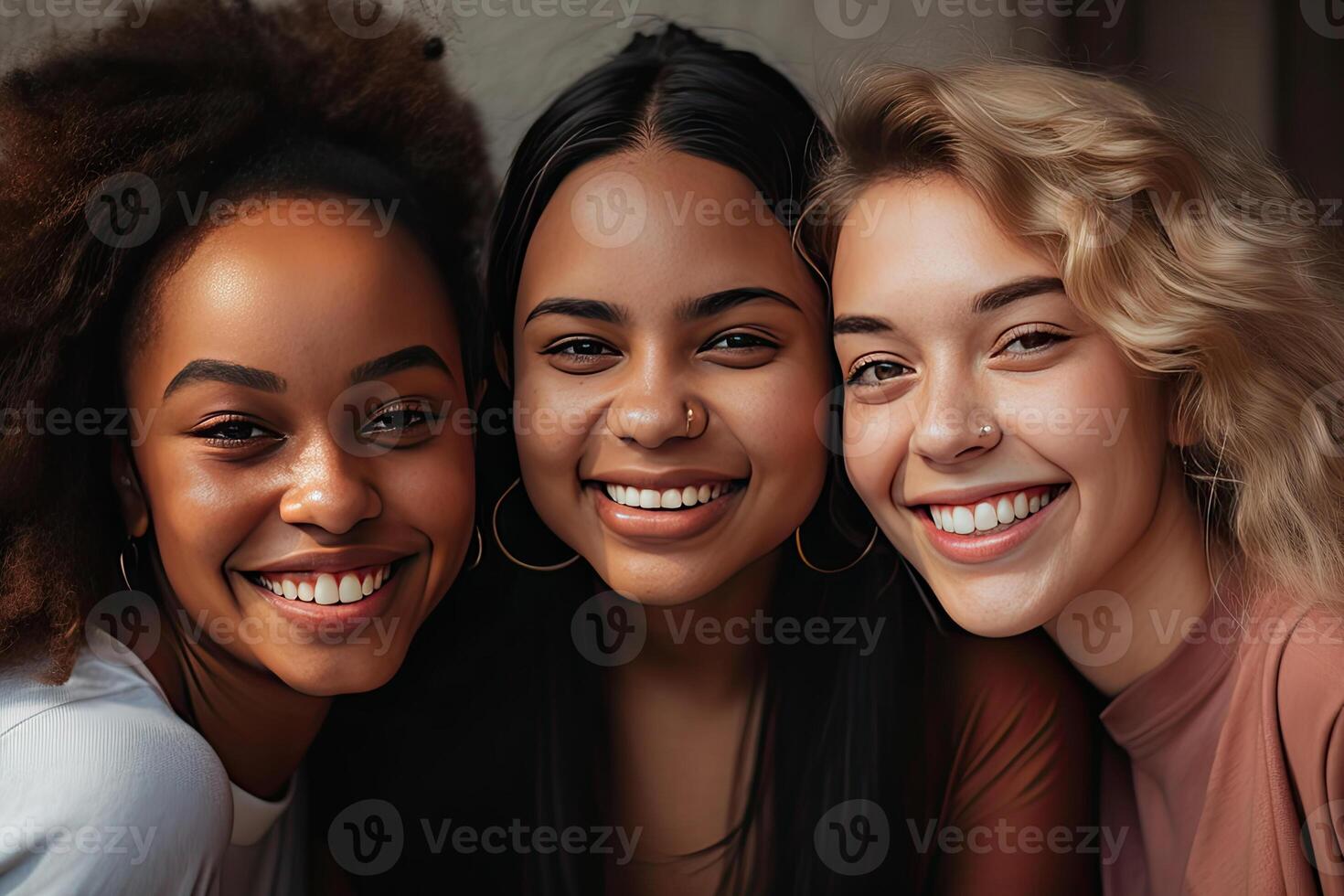
Benchmarking Fairness
To evaluate the current models for their difference and contextual awareness, a benchmark suite comprising various scenarios and multiple-choice questions was developed. The scenarios were categorized as descriptive (fact-based) or normative (value-based) to assess the models' performance in differentiating between groups appropriately.
Key Findings
The study revealed that existing state-of-the-art LLMs struggle with difference awareness and that common debiasing techniques may exacerbate the problem rather than solve it. Even models deemed fair by traditional benchmarks showed shortcomings when evaluated based on difference awareness metrics.

Additionally, prompt-based approaches aimed at mitigating bias were found to have negative effects on difference awareness, further emphasizing the need for a more nuanced understanding of fairness in AI systems.
Embracing Difference Awareness
Measuring and mitigating difference awareness in AI systems require a comprehensive consideration of the context in which these systems operate. It involves weighing the potential impact of treating demographic groups differently and determining whether such differentiation is necessary to address historical injustices and promote equity.
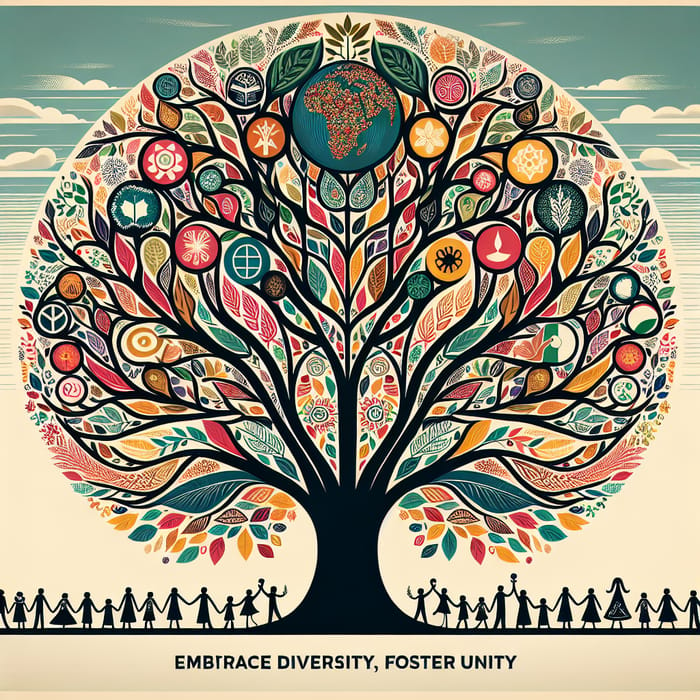
The findings underscore the importance of incorporating difference awareness into the development and evaluation of AI models to ensure a more inclusive and equitable technological landscape.