AlphaFold 3: Google DeepMind's latest AI tech in drug discovery
Google DeepMind has introduced its latest AI tech, AlphaFold 3. It is an AI model capable of accurately predicting the 3D folded structure of proteins based only on their amino acid sequences. AlphaFold can predict how proteins interact with one another and with other molecules, including DNA, RNA, and other small biomolecules.
Advancements in Protein Structure Predictions
AlphaFold 3 can accurately predict the intricate structures and interactions of biomolecules. This is a big step forward from AlphaFold 1 and AlphaFold 2, helping to understand proteins much better. AlphaFold 3 figures out how the protein interacts with other small molecules (ligands), DNA, RNA, and other proteins with remarkable accuracy rates compared to any other existing AI-developed model. Its true value lies in its ability to translate the shapes of these molecules into useful information about how they work and behave.
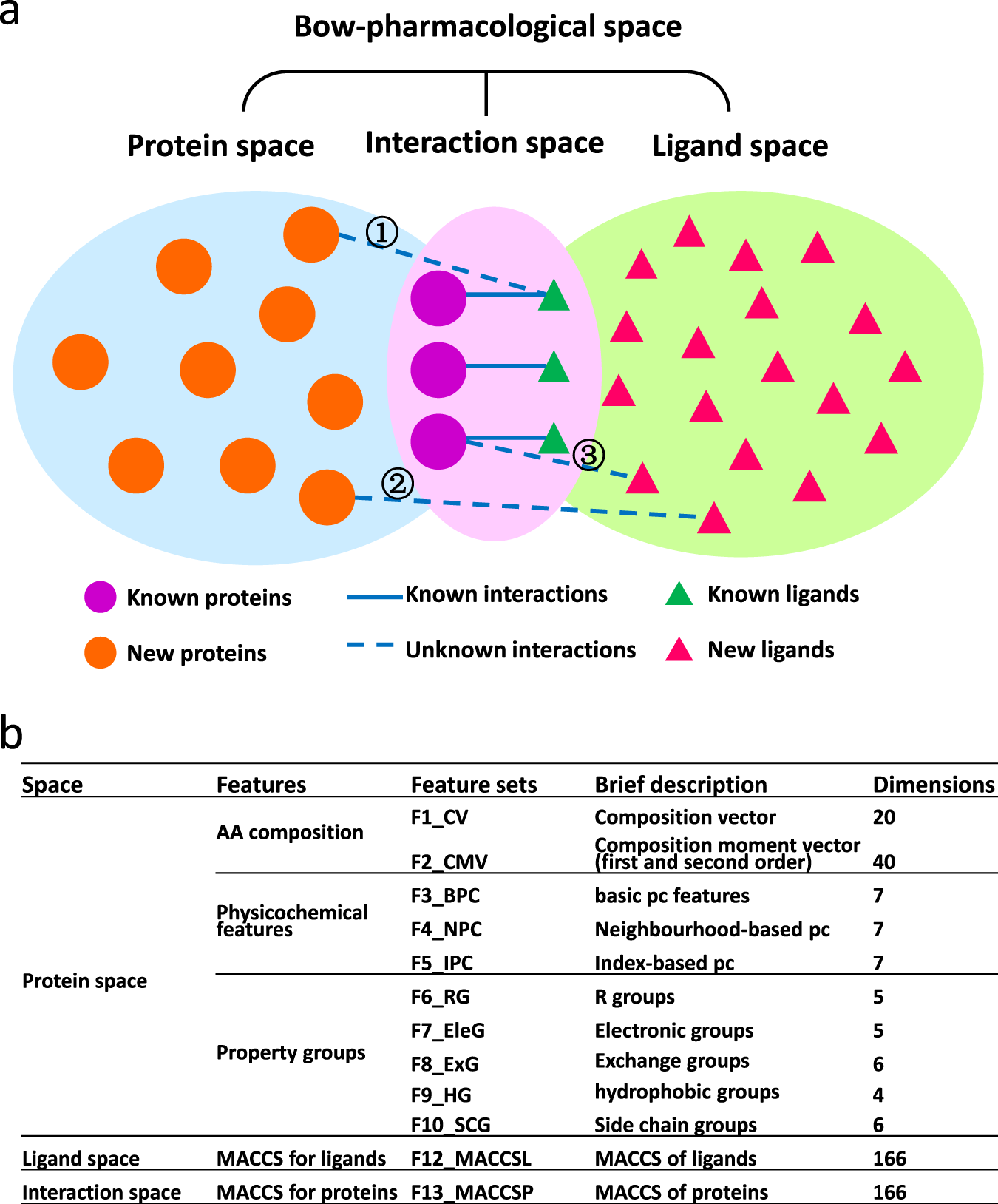
Google DeepMind has made AlphaFold Server openly accessible to make it easier for researchers worldwide to use AlphaFold 3. This approach is expected to drive breakthroughs in drug discovery, biotechnology, genomics, and our fundamental understanding of biological systems. However, unlike its previous models, DeepMind has not released the downloadable code of AlphaFold 3.
Importance of Protein Structure
Proteins are made of long chains of amino acids, which fold and twist to form 3D structures. This process is known as protein folding. Each protein follows a unique pattern of folding. So, a “3D protein structure” refers to the specific shapes that proteins adopt through folding.
A protein’s 3D structure will determine how a protein will interact with other molecules and perform its function. If a protein folds incorrectly or adopts an abnormal shape, it will not function properly, leading to degenerative diseases.
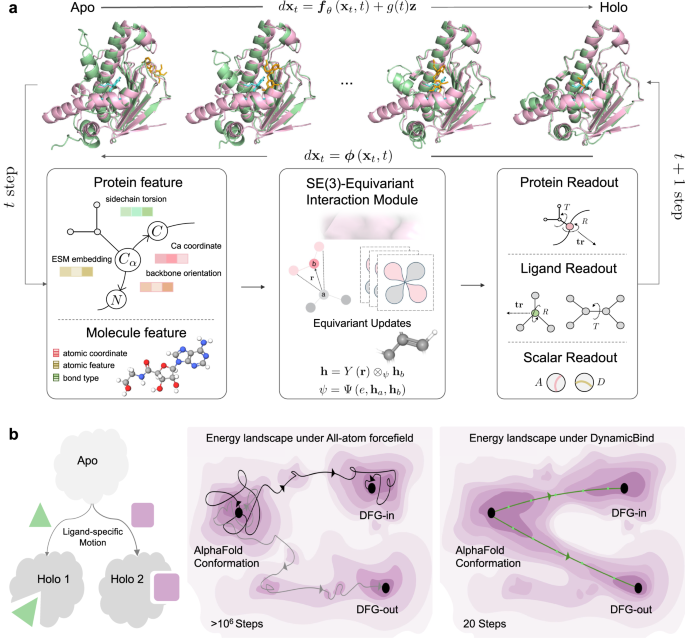
A protein’s 3D structure is the key to deciphering its molecular function and unraveling disease mechanisms. It also guides drug discovery efforts, explores evolutionary relationships, and enables protein engineering applications. Without protein’s structural information, understanding proteins and their roles in biological systems would be limited.
Applications in Drug Discovery
AlphaFold 3 can identify protein’s 3D structures without the meticulous trial and error that entails years of painstaking labor and costly financial investment. AlphaFold 3’s capability to predict protein-ligand interactions is essential for drug discovery. It enables precise modeling of how potential drug molecules bind to their target proteins, facilitating the identification and optimization of therapeutic candidates.
Understanding protein-nucleic acid interactions plays a relevant role in how genes are regulated. Accurate modeling of these interactions can provide essential insights into fundamental biological mechanisms and disease pathways.
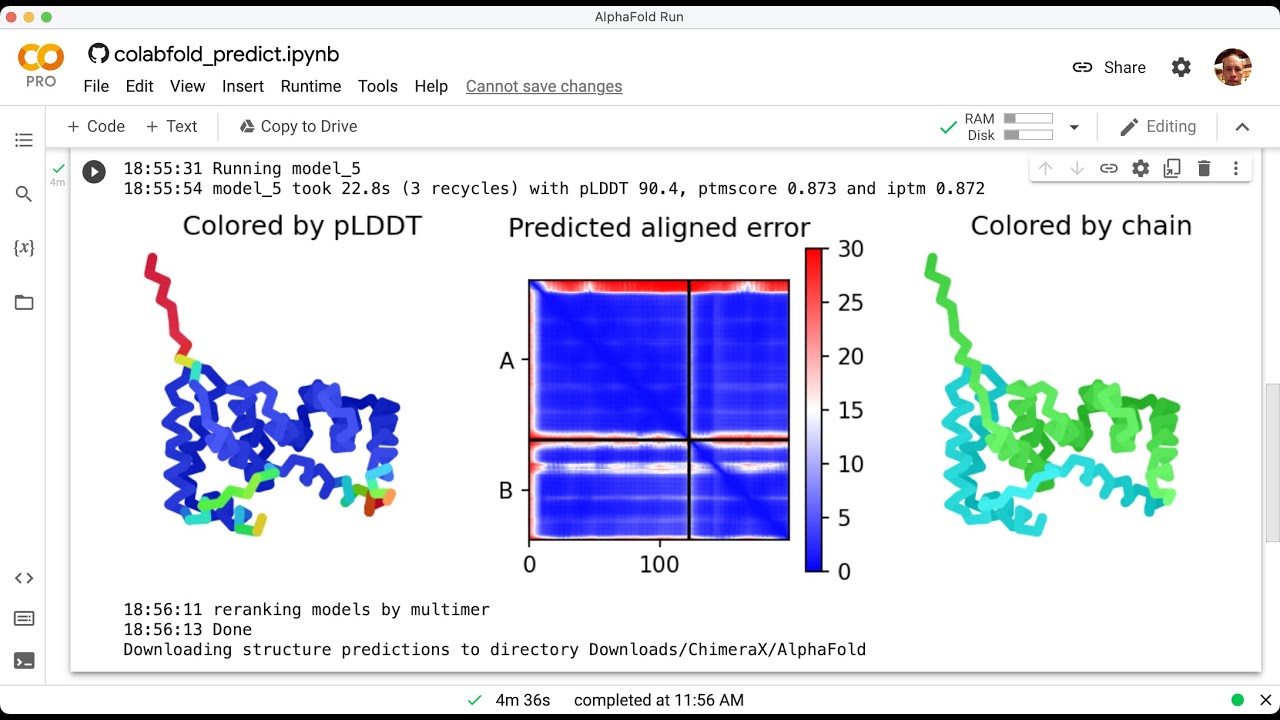
AlphaFold 3 has significantly enhanced prediction accuracy for antibody-antigen interactions. Learning about these interactions is essential for applications in immunology and therapeutic antibody development, helping to understand the exact binding between antibodies and antigens.
AlphaFold's Approach and Database Expansion
AlphaFold’s approach to predicting protein structures blends two key methods: bioinformatics and physics. The AI models use a physical and geometric inductive bias to learn from protein structure data without relying heavily on handcrafted features. AlphaFold can handle challenging cases like proteins with missing physical context or structures, producing accurate models with underspecified structural conditions present in public repositories of protein data.
The AlphaFold Database has expanded its collection of predicted protein structures by over 714 times, including over 214 million structures, up from the initial 300,000 in 2021. The impact is enormous, helping in finding new drugs, understanding diseases better, and improving biotechnology across various industries.
Contributions to Drug Discovery
AlphaFold has the potential to tackle world problems beyond drug discovery, such as plastic pollution. It has been utilized to design new types of enzymes that can more efficiently break down plastic waste, offering sustainable solutions for recycling. The AI model's applications extend to biofuel production and improving food production processes, making them more efficient and economically viable.
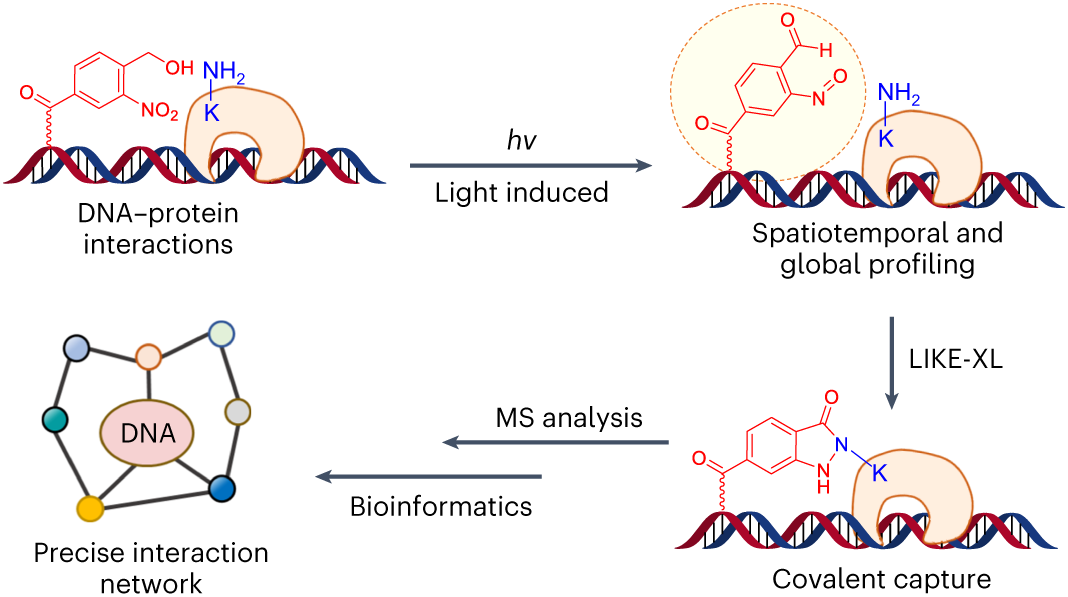
Undoubtedly, AlphaFold’s impact is already palpable across industries, prompting consideration of its potential applications. It enhances drug development processes and leads to potential new treatments for various diseases.