Why Human-Created Input Data Is Needed to Maintain AI Models...
When it comes to training Generative AI models, the data used plays a crucial role in the model's performance. While a significant amount of data for AI models is sourced from the internet and various other channels, the quality of the data matters. With Gen AI being leveraged to produce vast volumes of new content that is readily available online, the question arises - what happens when this AI-generated content is looped back into the model as additional training data?
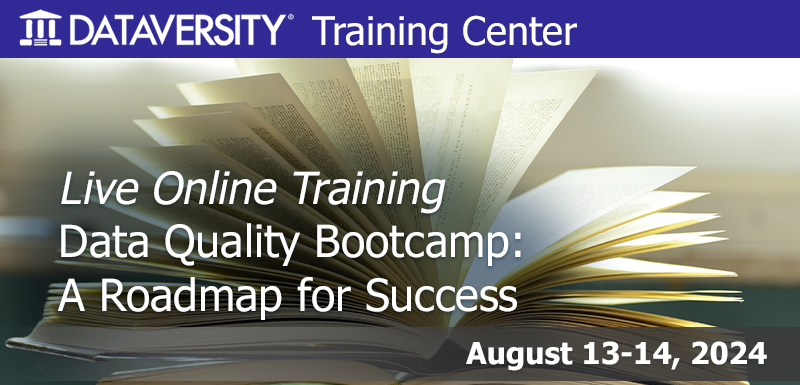
At first glance, one might assume that incorporating Gen AI outputs as training data would only serve to strengthen the model, especially considering the fluency with which AI-generated content mimics human-created content. However, recent research led by Oxford professor Ilya Shumailov has unearthed some alarming findings. It appears that even a small infusion of AI-generated data back into the model can trigger what is referred to as "model collapse."
The Impact of AI-Generated Data on Model Training
The essence of the issue lies in how Generative AI functions. While naturally occurring training data typically presents a broad distribution, Gen AI tends to produce outputs that align with the most probable choices, essentially favoring data from the middle of the bell curve. This tendency results in the over-representation of common data points, as illustrated by the example of a dataset featuring various dog breeds.
As the AI model iteratively processes Gen AI outputs as new training data, the prevalence of certain data points, such as the golden retriever breed in the aforementioned example, increases significantly. This overemphasis eventually distorts the original training data, leading to a loss of coherence in the model's outputs. Instead of maintaining the characteristics of the initial data, the model starts generating nonsensical results, deviating from the expected outputs.
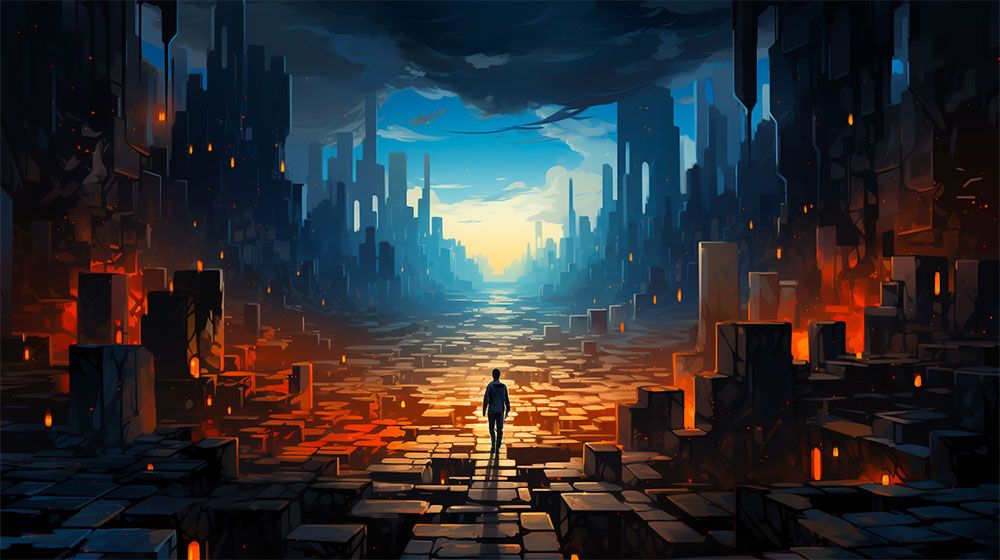
Addressing Model Collapse and Ensuring Data Quality
The phenomenon of model collapse, while concerning, has sparked discussions in the AI community regarding the importance of maintaining a balance between synthetic and human-created training data. Some researchers argue that the adverse effects of model collapse can be mitigated through a strategic mix of data sources, safeguarding the model's integrity and performance.
A notable response to the risks posed by the reuse of Gen AI outputs has been the advocacy for regulatory measures, such as the proposed California law AB3211, which seeks to mandate watermarking on AI-generated content. By implementing watermarks on such content, not only can consumers be informed about the origin of the data, but it also offers a practical solution for distinguishing and excluding LLM-generated content during data collection processes.
Conclusion
As the AI landscape continues to evolve, ensuring the quality and integrity of training data is paramount for maintaining the efficacy and reliability of AI models. By understanding the implications of incorporating AI-generated data into model training, researchers and practitioners can work towards enhancing the performance and resilience of AI systems in the long run.
For further insights on AI-related topics and legal considerations, you can explore upcoming events like:
- The EU AI Act - An Introduction to Risks, Compliance & More - Learn Live
- Copyright Protection - Digging into The Detail - Learn Live
- ChatGPT & Generative AI - The Legal & Ethical Issues - Learn Live
- Indecent Images, Deep Fakes & AI - What Criminal Lawyers Need to Know - Learn Live
- Copyright - Recent Developments & Lessons Learned - Learn Live
© Copyright 2006 - 2024 Law Business Research