Meta AI Introduces MILS: A Training-Free Multimodal AI Framework
Large Language Models (LLMs) are primarily designed for text-based tasks, limiting their ability to interpret and generate multimodal content such as images, videos, and audio. Conventionally, multimodal operations are task-specific models trained on large amounts of labeled data, which makes them resource-hungry and rigid. Zero-shot methods are also restricted to pretraining with paired multimodal datasets, limiting their flexibility to new tasks. The challenge is to make LLMs perform multimodal reasoning and generation without task-specific training, curated data, or model adaptation. Overcoming this challenge would significantly enhance the applicability of LLMs to multimodal content processing and generation dynamically across multiple domains.
Challenges of Existing Multimodal AI Systems
Conventional multimodal AI systems are based on models like CLIP for image-text alignment or diffusion models for media generation. Still, these methods are restricted to extensive training on curated data. Zero-shot captioning models like ZeroCap and MeaCap try to overcome this but are still restricted to fixed architectures and gradient-based optimization, restricting their generalization capability across different modalities.
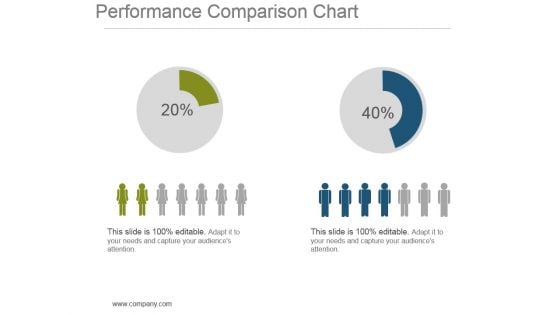
These methods have three limitations: they are restricted to extensive labeled data, they cannot generalize beyond the training distribution, and they are based on gradient-based methods that restrict their flexibility to new tasks. Without overcoming these limitations, multimodal AI is restricted to fixed tasks and datasets, restricting its potential for further applications.
Introducing MILS by Meta
Researchers from Meta propose MILS (Multimodal Iterative LLM Solver), a test-time optimization framework that enhances LLMs with multimodal reasoning capabilities without requiring additional training. MILS uses an iterative optimization cycle with a GENERATOR and a SCORER. The GENERATOR, an LLM, produces candidate solutions for multimodal tasks like image captions, video descriptions, or stylized image prompts, while the SCORER, a pre-trained multimodal model, ranks the generated solutions by relevance, coherence, and alignment with input data. Alternating between the two, MILS repeatedly refines its outputs with real-time feedback, continually improving performance.
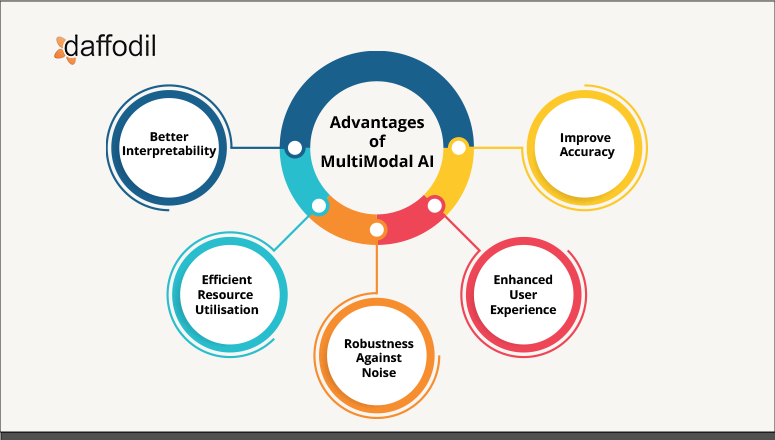
This enables zero-shot generalization across several modalities, including text, images, videos, and audio, making it an extremely versatile solution for multimodal AI applications.
Implementation and Performance
MILS is implemented as a gradient-free optimization method, employing pre-trained models without tuning their parameters. The framework has been used in a variety of multimodal tasks. For image captioning, MILS employs Llama 3.1 8B as the GENERATOR and CLIP-based models as the SCORER to iteratively find optimal captions until the most accurate and descriptive caption is generated.
MILS achieves robust zero-shot performance on a variety of multimodal tasks and outperforms previous work on both captioning and generation. For image captioning, it is more semantically accurate than previous zero-shot models and generates more natural and informative captions.
Further Advancements and Applications
Using pre-trained LLMs and multimodal models in adaptive feedback, MILS creates a new state-of-the-art for multimodal AI, allowing for more adaptive and scalable AI systems that can dynamically process multimodal reasoning and generation tasks.
For more information, you can check out the Paper and GitHub Page. All credit for this research goes to the researchers of this project.