Evaluating ChatGPT for structured data extraction from clinical notes
In a recent study published in npj Digital Medicine, researchers evaluated ChatGPT's ability to extract structured data from unstructured clinical notes.
Large-language-based models (LLMs), including Generative Pre-trained Transformer (GPT) artificial intelligence (AI) models like ChatGPT, are used in healthcare to improve patient-clinician communication. Traditional natural language processing (NLP) approaches like deep learning require problem-specific annotations and model training. However, the lack of human-annotated data, combined with the expenses associated with these models, makes building these algorithms difficult.
Thus, LLMs like ChatGPT provide a viable alternative by relying on logical reasoning and knowledge to aid language processing. In the present study, researchers create an LLM-based method for extracting structured data from clinical notes and subsequently converting unstructured text into structured and analyzable data. To this end, the ChatGPT 3.50-turbo model was used, as it is associated with specific Artificial General Intelligence (AGI) capabilities.

Data Transformation and Analysis
A total of 1,026 lung tumor pathology reports and 191 pediatric osteosarcoma reports from the Cancer Digital Slide Archive (CDSA), which served as the training set, as well as the Cancer Genome Atlas (TCGA), which served as the testing set, were transformed to text using R program. Text data was subsequently analyzed using the OpenAI API, which extracted structured data based on specific prompts.
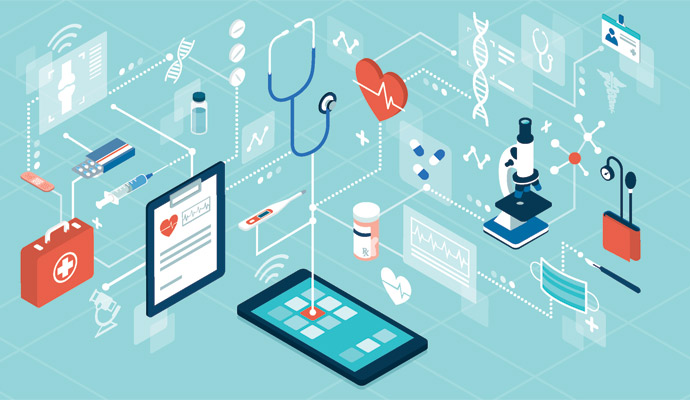
ChatGPT API was used to perform batch queries, followed by prompt engineering to call the GPT service. Post-processing involved parsing and cleaning GPT output, evaluating outcomes against reference data, and obtaining feedback from domain experts. These processes aimed to extract information on TNM staging and histology type as structured attributes from unstructured pathology reports.
Model Performance Evaluation
From the 99 reports acquired from the CDSA database, 21 were excluded due to low scanning quality, near-empty data content, or missing reports. This led to a total of 78 genuine pathology reports used to train the prompts. To assess model performance, 1,024 pathology reports were obtained from cBioPortal, 97 of which were eliminated due to overlapping with training data.
ChatGPT was directed to utilize the seventh edition of the American Joint Committee on Cancer (AJCC) Cancer Staging Manual for reference. Data analyzed included primary tumor (pT) and lymph node (pN) staging, histological type, and tumor stage. The performance of ChatGPT was compared to that of a keyword search algorithm and deep learning-based Named Entity Recognition (NER) approach.
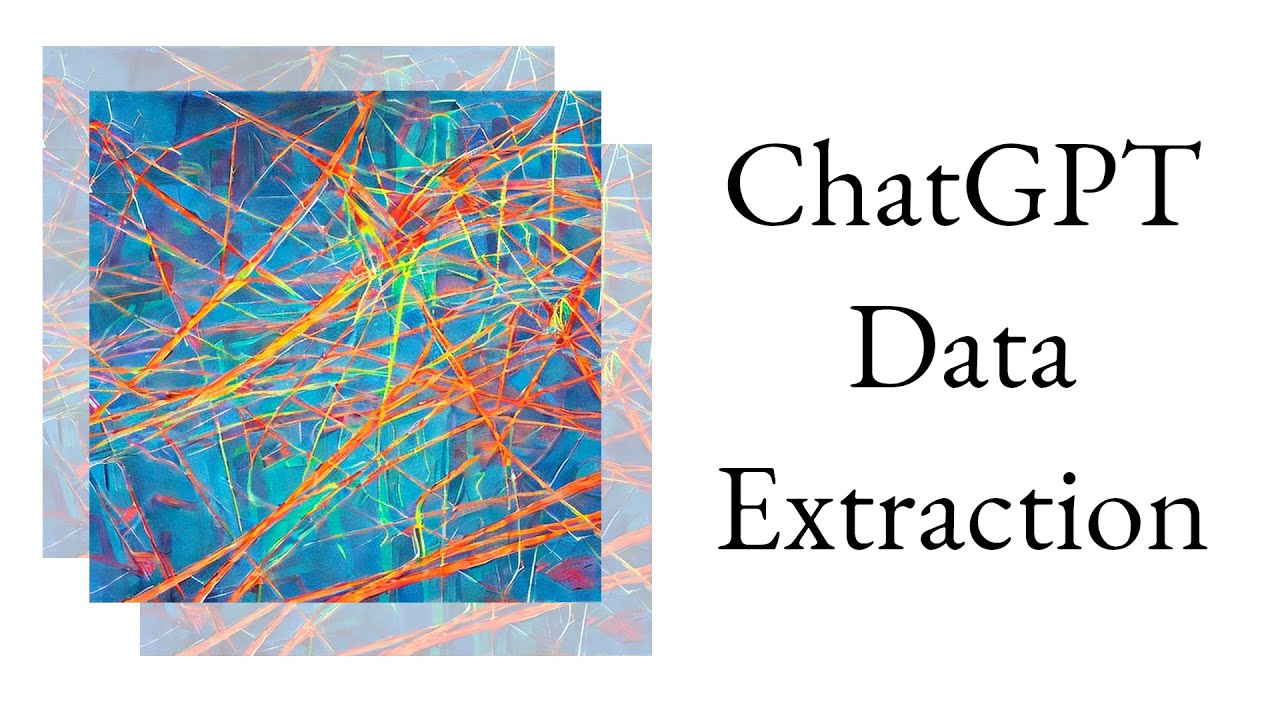
Key Findings
A detailed error analysis was conducted to identify the types and potential reasons for misclassifications. ChatGPT version 3.50 achieved 89% accuracy in extracting pathological classifications from the lung tumor dataset, outperforming other algorithms. It also accurately classified grades and margin status in osteosarcoma reports, with an accuracy rate of 98.6%.
However, model performance was affected by the instructional prompt design, leading to misclassifications and improper interpretations. ChatGPT-3.50 also showed consistent performance over time but had challenges in certain categories.
Conclusion
ChatGPT appears to be capable of handling massive clinical note volumes to extract structured data without requiring considerable task-based human annotation or model data training. This study highlights the potential of LLMs to convert unstructured healthcare information into organized representations, facilitating research and clinical decisions in the future.
Posted in: Device / Technology News | Medical Research News | Healthcare News
Tags: Artificial Intelligence, Cancer, Deep Learning, Genome, Healthcare, Histology, Language, Lymph Node, Medicine, Osteosarcoma, Pathology, Propagation, Research, Tumor