ChatGPT-4o can technically run Linear Mixed Models — but I don't ...
As I venture through the assignments in my graduate level biostatistics course, we encounter models and methods of increasing complexity, posing more challenges to ChatGPT-4o. Recently, we explored its ability (or lack thereof) to run Generalized Additive Models. This week, the focus shifted to evaluating its capability to handle Linear Mixed Models.
Understanding Linear Mixed Models
Mixed models represent a specific category of regression models that incorporate both fixed and random effects. When it comes to statistics, we typically consider fixed effects as predictors with a discernible impact on the response variable. On the other hand, random effects account for variables related to the response, where the focus lies more on mitigating bias rather than estimating specific impacts. These concepts are essential for students working on their thesis projects, where such models are commonly employed for data analysis.
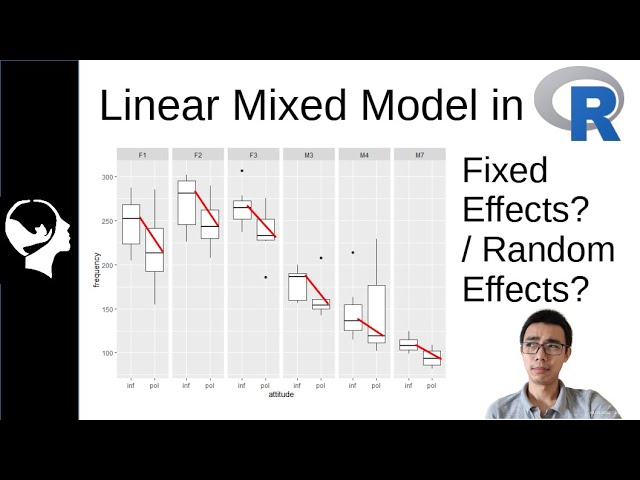
Practical Applications of Linear Mixed Models
One classic example I often share with my students pertains to educational studies. Imagine we're assessing the efficacy of a new teaching method on student groups. While it might seem straightforward to compare the performance of students under the new method versus the traditional one, there's a crucial factor to consider—variations in teaching effectiveness among different educators.

Ignoring this variability could lead to pseudoreplication. In such cases, including teachers as fixed effects might not be ideal as it could significantly impact statistical power. Instead, by treating teachers as random effects, assuming their effectiveness follows a normal distribution, we can effectively incorporate this variability into our analysis without directly comparing individual teachers.
Teaching and Demonstrating
In my instructional sessions, I delve into linear models with increasing intricacy, exploring interactions, main effects, and the nuances of mixed models with random intercepts and slopes. Utilizing the nlme package in R, we fit these models, decode summary outputs, and interpret diagnostic plots. Practical exercises further reinforce these concepts, guiding students to apply similar models independently.
Evaluating ChatGPT-4o's Performance
For the latest assignment, I tasked ChatGPT-4o with conducting linear regressions using real data, specifically focusing on the relationship between different variables. While the model could successfully fit basic linear regressions, it stumbled when challenged with more complex interactive models. Despite minor discrepancies in results compared to traditional statistical tools like R, ChatGPT-4o demonstrated the potential to handle statistical analyses, albeit with room for improvement.
As we navigate the realm of statistical modeling, the role of AI tools like ChatGPT-4o in supplementing traditional methodologies continues to evolve, offering both opportunities and challenges in the field of statistical analysis.