A Step Towards A New Generation of Multimodal Models in AI ...
In a world with various types of data such as pictures, text, sounds, and more, multimodal models have emerged as the polyglots of AI, mimicking the brain's ability to process multiple inputs simultaneously. These intricate neural systems amalgamate diverse information into a unified system, bridging the gaps between different types of data.
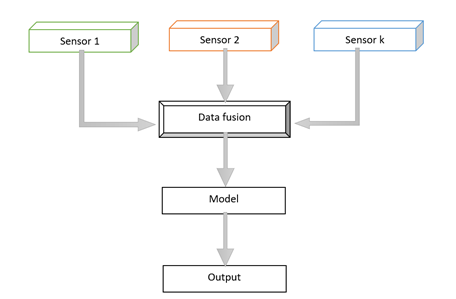
By the year 2025, a multitude of enterprise applications are projected to incorporate AI features with multimodal capabilities, addressing the grand challenge of capturing and processing multiple types of information simultaneously. Multimodal models are poised to play a pivotal role in solving complex real-world problems, offering a more nuanced understanding of data.
Importance of Multimodal Models
MMI models have garnered significant attention in the realms of AI and machine learning, leading to advancements across various industries. These models provide additional context that influences decision-making in sectors ranging from healthcare to entertainment and even e-commerce.
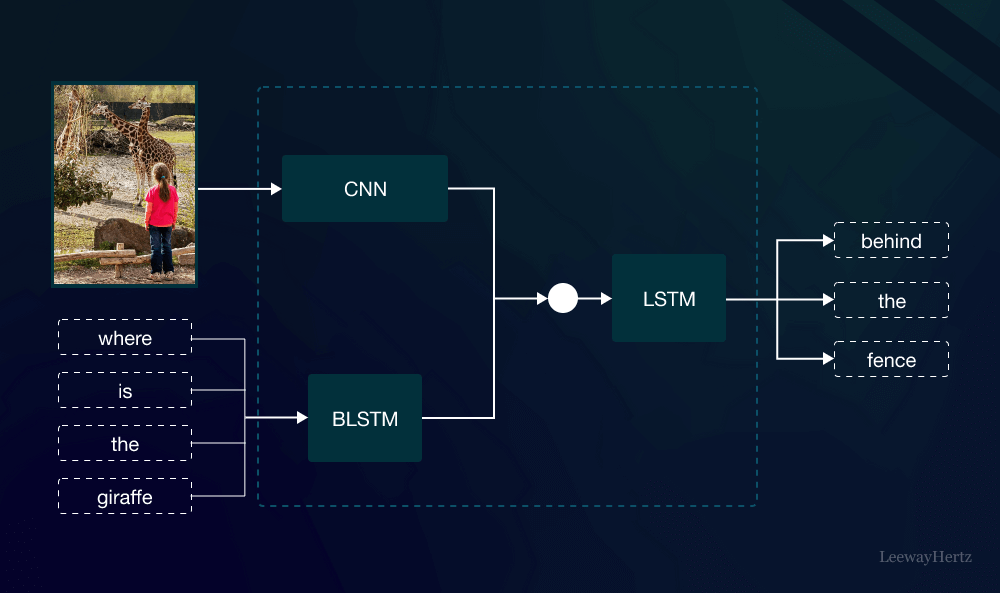
A multimodal model is designed to process data input and output across various modes or modalities, such as written text, images, sound, and video. By encompassing a wide array of features during training, multimodal models enhance the comprehension of complex information, resulting in more precise and detailed outputs.
Evolution of Multimodal Models
Initially, AI models were tailored to handle a single type of data, like text-based models for natural language processing (NLP) or image recognition models. However, the inadequacy of single-modal models in comprehending complex information prompted researchers to explore the fusion of different data types to enhance AI capabilities.
Technological advancements like Convolutional Neural Networks (CNNs) and Transformers have revolutionized multimodal models by enabling the amalgamation of visual, textual, and auditory data for improved performance in various tasks.
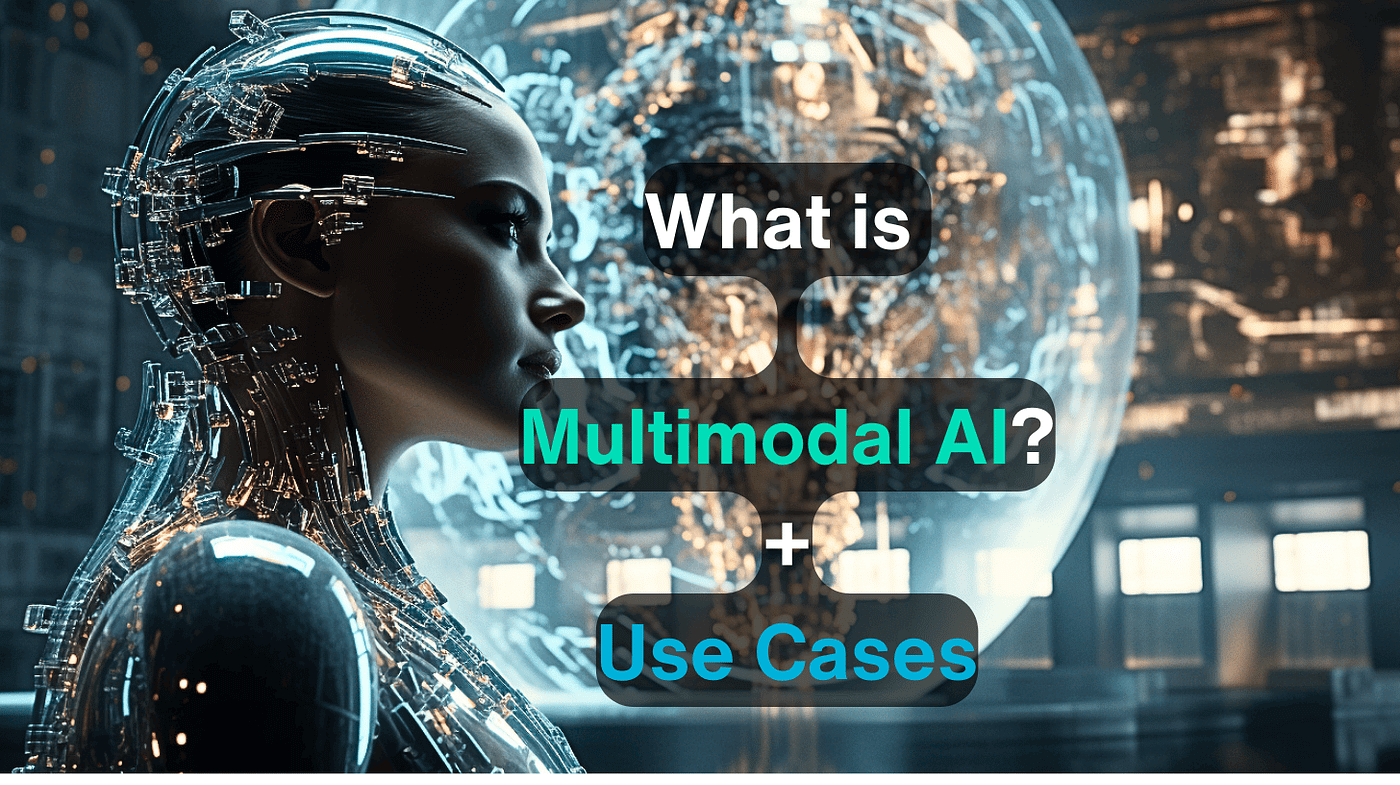
Technological Innovations in Multimodal Models
Deep learning techniques, such as CNNs and Recurrent Neural Networks (RNNs), are instrumental in handling multi-modal data fusion. The advent of Transformers, popularized by models like BERT and GPT, has further augmented the processing of diverse information types within multimodal models.
Data fusion techniques play a crucial role in integrating information from different sources, while cross-modal learning facilitates understanding relationships between modalities. These advancements empower multimodal models to deliver more accurate and comprehensive outputs, particularly in tasks requiring high-level understanding.
Applications of Multimodal Models
Multimodal models find applications beyond translation, offering enhanced user experiences in entertainment, customer service chatbots, and healthcare diagnostics. These models enable seamless integration of different input/output modes, fostering natural user-machine interactions and adaptable learning capabilities.
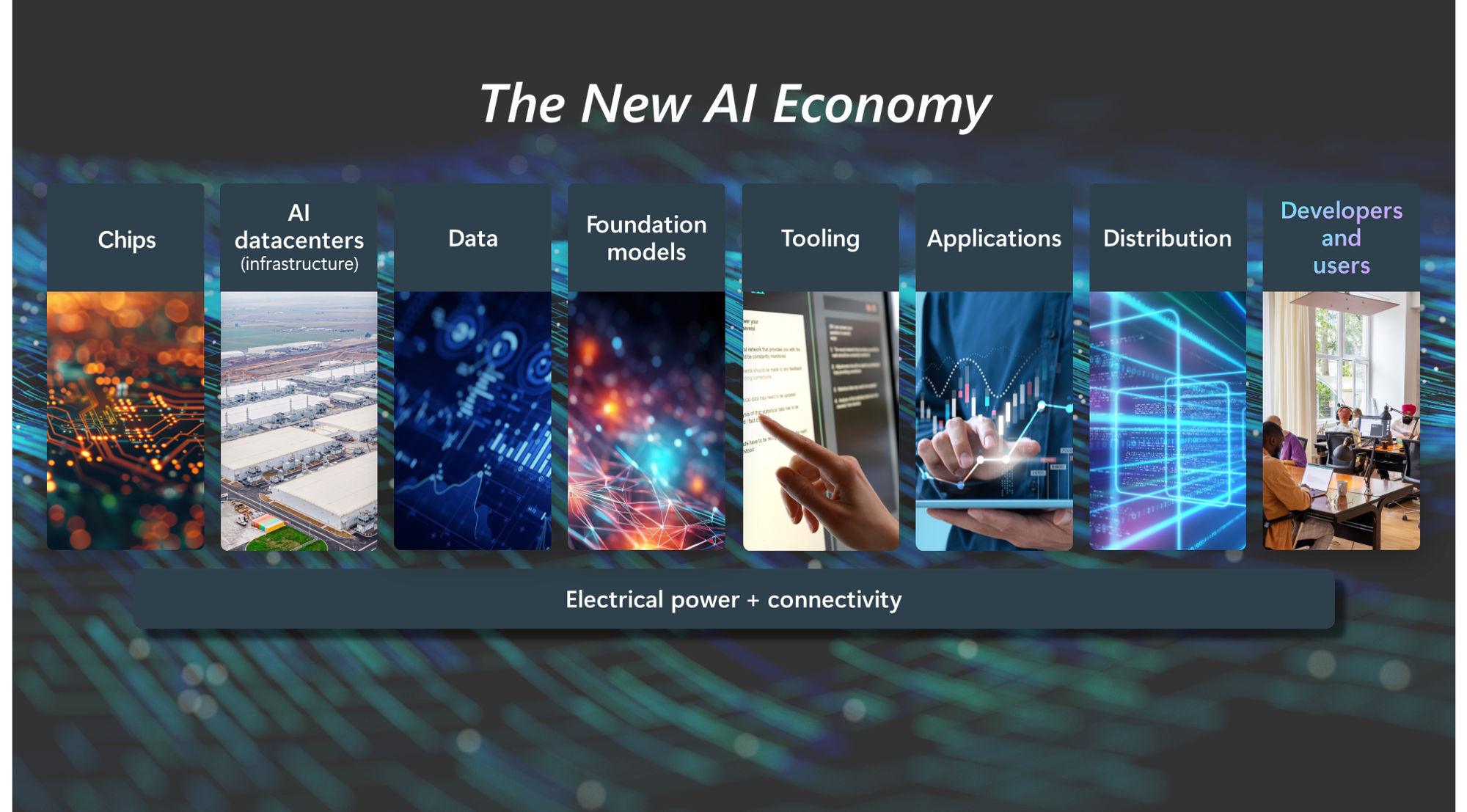
Leading tech companies like Google and Amazon leverage multimodal AI systems for diverse applications, from healthcare decision-making to autonomous driving technology. By harnessing the power of multimodal models, businesses can cater to evolving needs and maximize the value of their technology investments.