HuatuoGPT-o1: Advanced Medical Reasoning by AI on Air
HuatuoGPT-o1 is a cutting-edge large language model (LLM) tailored specifically for advanced medical reasoning. This revolutionary AI tool is designed to enhance medical diagnostics and treatment planning, showcasing its potential applications in the healthcare industry. The focus lies on the exceptional capabilities of HuatuoGPT-o1 in navigating complex medical decision-making processes, marking a significant leap forward in AI-driven healthcare solutions.
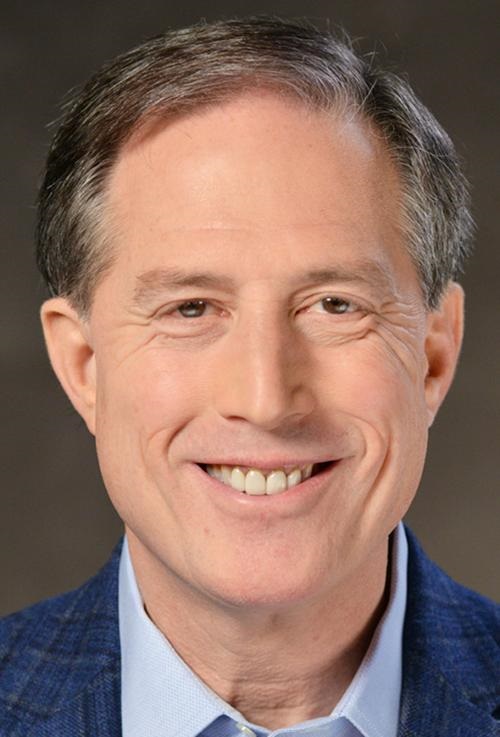
Advancements in AI Healthcare Technology
Sana recently shared an article highlighting the FDA's approval of Sepsis ImmunoScore, an AI-powered tool dedicated to early sepsis detection. This milestone approval signifies a major breakthrough in the realm of AI's role in healthcare diagnostics, making it the first AI tool to receive such recognition from the FDA. The growing acceptance of AI technology in healthcare, coupled with increased regulatory oversight, underlines a pivotal evolution in the field of medical AI implementation and regulation.
Commitment to Safety and Efficiency
OpenAI researchers have introduced a set of guidelines aimed at enhancing the safety, accountability, and efficiency of advanced AI systems. These guidelines serve to address critical challenges in the responsible development and deployment of potent AI technologies, ultimately providing a framework for constructing more secure and dependable AI systems.
Industry Trends and Innovations
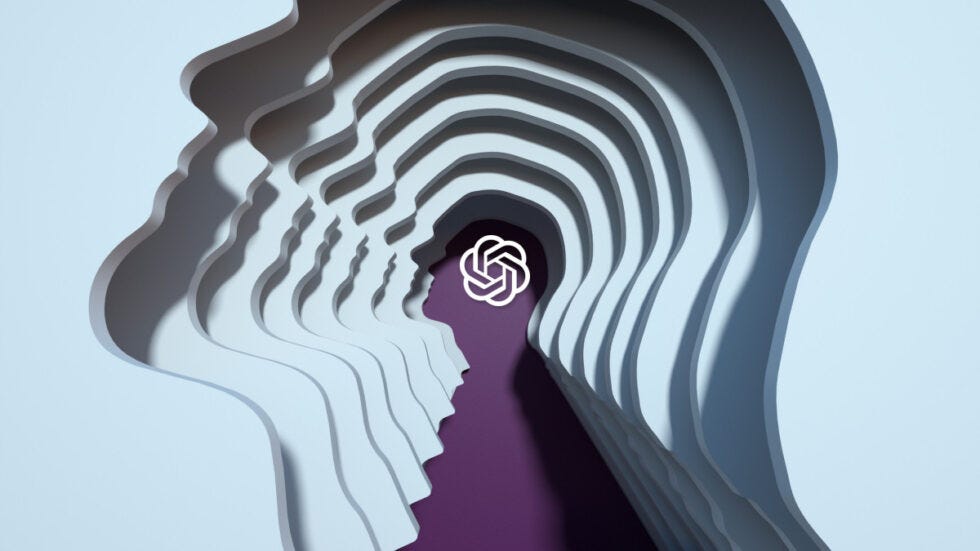
The episode delves into Apple's strategic foray into the competitive AI market, emphasizing its privacy-centric, on-device approach in contrast to rivals like Microsoft and OpenAI. This move reflects broader industry trends indicating a shift towards more sophisticated AI capabilities, including emotional AI. Apple's unique positioning in the AI landscape signals its intent to carve out a distinctive niche in the ever-evolving AI sector.
Transformative Techniques in AI Development

Mix-LN, a novel normalization technique for transformer architectures, strikes a balance between training stability and performance, showcasing improved convergence without compromising model quality. This hybrid approach has demonstrated success across various applications, such as machine translation and language modeling, addressing a key challenge in transformer model advancement.