Army faces data overload but LLMs are not the answer
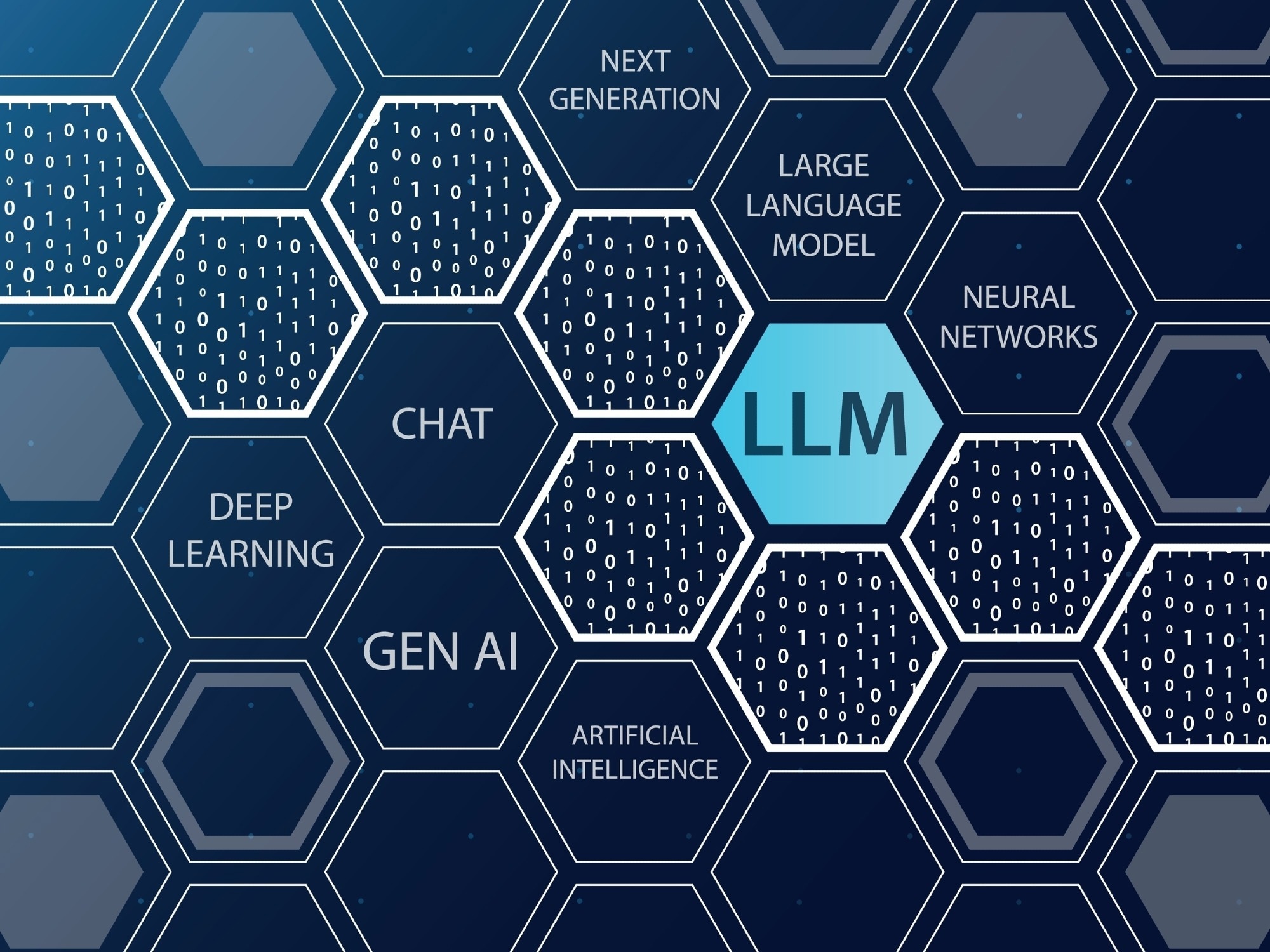
Army leaders and soldiers are facing a significant challenge - being inundated with vast amounts of data. The sheer volume of information is overwhelming, hindering decision-making processes and leading to analysis paralysis. While it may seem tempting to turn to advanced tools like Chat GPT for solutions, Stephen Riley warns that this might not be the answer.
Riley, a member of the Army engineering team at Google, emphasized the limitations of utilizing large language models (LLMs) such as Chat GPT or Gemini for military applications. He highlighted the impracticality of relying on such resource-intensive tools, especially at the tactical edge where compute resources are scarce.
The Army, being one of the largest AI users among military branches, generates a massive amount of data due to its extensive operations and personnel. However, simply having access to this data does not guarantee actionable insights for Army leaders.
Effective Alternatives to LLMs
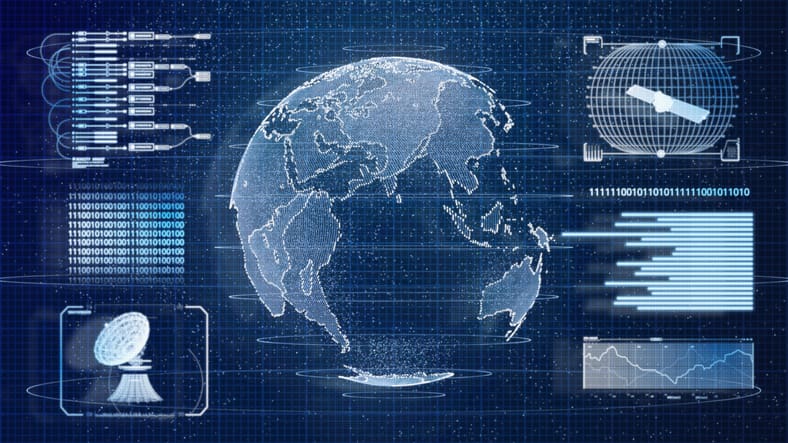
Instead of solely depending on LLMs, Riley suggested that the Army could adopt strategies similar to those employed by tech companies like Google. By focusing on improving search result quality through data analysis and the development of knowledge graphs representing established truths and relationships, the Army can streamline its data processing without the need for complex LLMs.
Riley proposed the creation of a knowledge graph specific to the Army, encoding essential information that can be accessed with minimal computational power. This method, while requiring some effort to implement, offers a more efficient and practical approach compared to deploying massive language models.
Combining AI Models for Better Results
Young Bang echoed Riley's sentiments, suggesting that a combination of large and small language models, along with knowledge graphs, could provide superior results for military applications. By overlaying a knowledge graph onto AI datasets, the military can enhance data processing capabilities without the need for excessive computational resources.
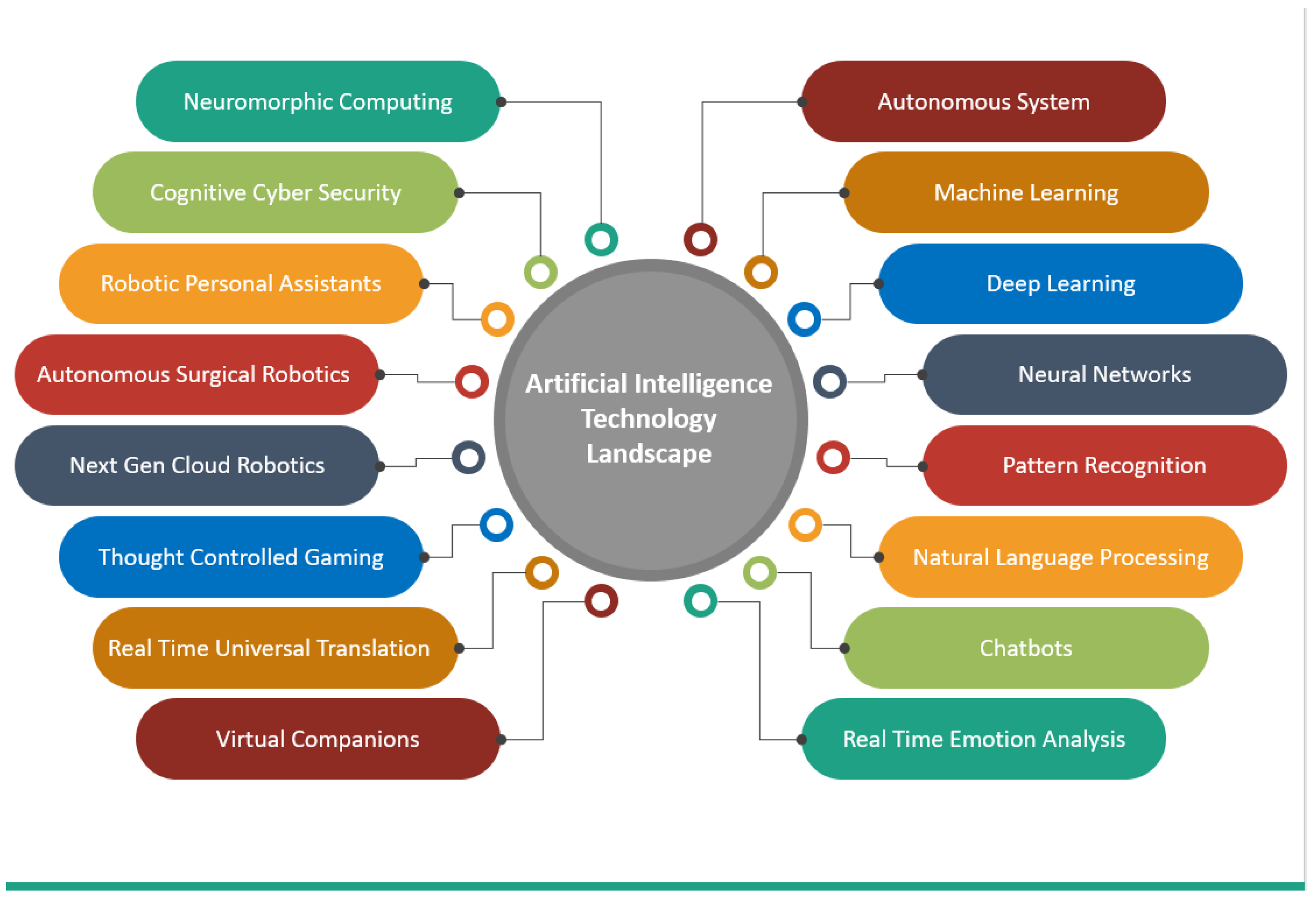
One of the primary concerns surrounding AI datasets is the potential for malicious actors to introduce misinformation, leading to a distortion of commonly accepted truths. Riley stressed the importance of human oversight in preventing such manipulations, emphasizing the role of human reasoning alongside machine learning algorithms.
Ensuring Data Integrity and Transparency
Both Riley and Bang emphasized the need for transparency in data sourcing, especially when acquiring AI models from commercial vendors. They urged military organizations to demand visibility into the origins of data to prevent the inadvertent integration of false or unethical information into training datasets.
By establishing a "data bill of materials" as a standard part of AI system acquisition, the military can mitigate the risks associated with tainted data and ensure the integrity of their AI applications.