Pavel Plutov on LinkedIn: #generativeai #enterpriseai #ibm ...
IBM has completed over 1,000 generative AI projects in just one year, showcasing the rapid adoption and impact of this technology across various business functions. Some key highlights include:
- Customer service automation with 95% accuracy
- Marketing personalization reducing costs by up to 40%
- 90% reduction in text analysis tasks for knowledge workers
- 50% reduction in employee mobility processing time
- Supply chain invoice costs cut by up to 50%
- 80% faster data processing for planning and analysis
- IT operations MTTR reduced by 50%
- Application support tickets cut by 70%
- Threat management response times reduced from hours to minutes
IBM's Open-Source Initiative:
IBM also open-sourced its Granite 13B LLM in May, optimized for enterprise use cases and outperforming comparable models on many tasks.
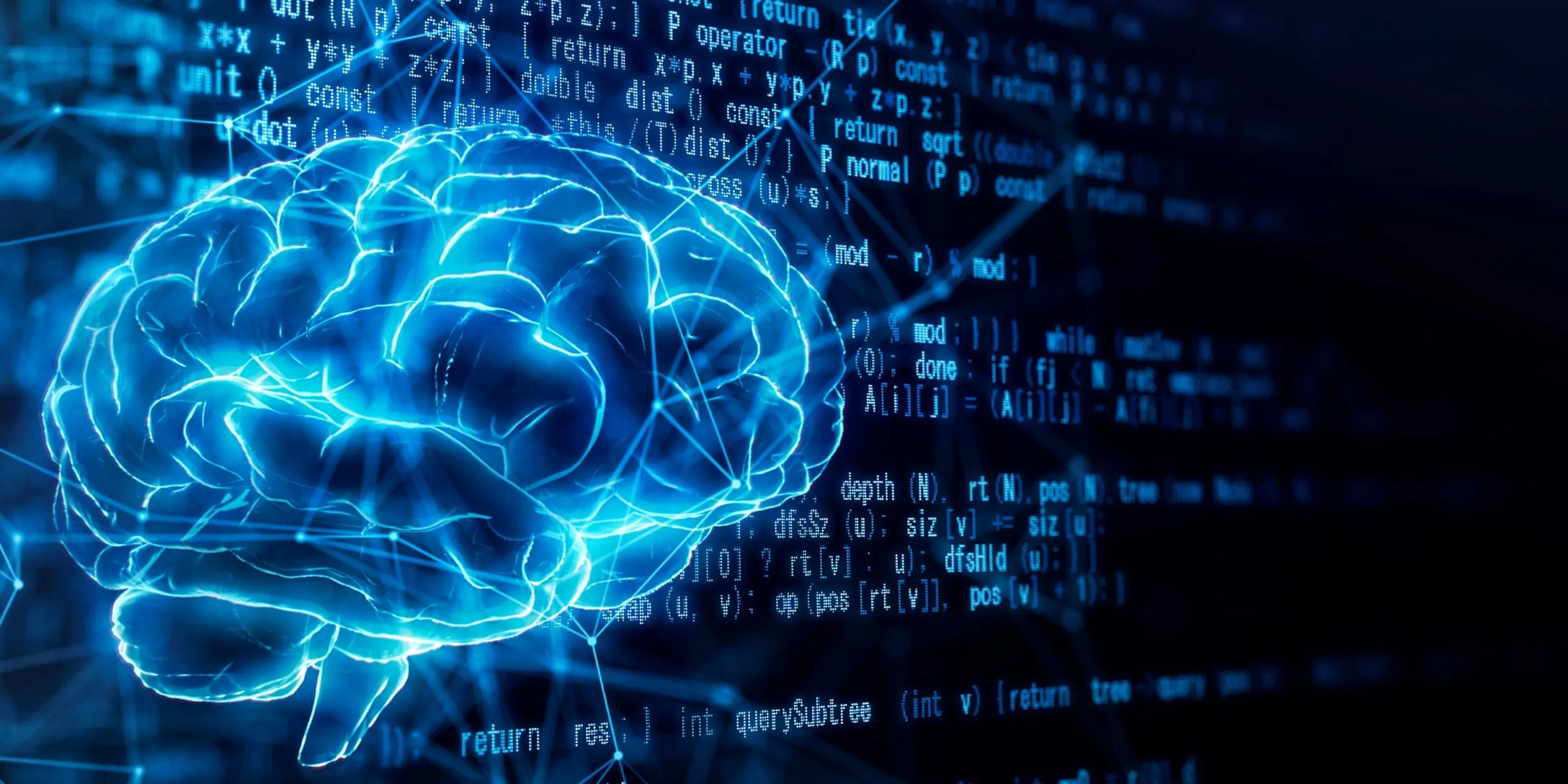
Spotlight on Israel:
Here in Israel, my group is conducting dozens of projects and POCs, particularly with startups and ISVs. Our main focus areas include enhancing usability, functionality, and customer experience of startups' commercial solutions, applications assistants interact with the application’s data and the UI through natural language, boosting knowledge worker productivity, and upgrading customer support. These AI implementations showcase how quickly generative AI is transforming business operations across industries and company sizes.

As more organizations adopt these technologies, we expect continued efficiency gains and new innovative use cases to emerge.
Fairness Alert: The Hidden Dangers in Synthetic Data for AI
As AI continues to reshape industries, there is a growing reliance on synthetic data to train and fine-tune models. However, there is a critical issue of fairness in synthetic data generation methods, which may not accurately represent all subgroups defined by protected attributes, leading to bias.
Key Points to Consider:
- Unfair synthetic data can lead to biased AI models, potentially amplifying societal inequalities.
- Even if your original data is balanced, synthetic data generation might introduce new biases.
- Fine-tuning AI models with biased synthetic data can propagate and exacerbate unfairness, even if the original model was unbiased.
- Detecting bias in synthetic data can be more challenging than in real data, increasing the risk of deploying unfair models.
The solution lies in implementing regular fairness KPIs evaluation during development, using diverse, real-world testing sets, continuously monitoring model outputs in production, establishing feedback loops to address fairness issues, and maintaining transparency about synthetic data usage.

IBM is actively addressing this challenge with #watsonx by implementing guardrails on model outputs, offering transparent models with #IBMGranite, and providing #watsonxgovernance to manage the full lifecycle of models from development to production.