Google's AlphaEvolve: The AI agent that reclaimed 0.7% of Google's Compute Capacity
Join our daily and weekly newsletters for the latest updates and exclusive content on industry-leading AI coverage. Learn MoreGoogle's new AlphaEvolve demonstrates the transition of an AI agent from a lab demo to practical application. Developed by Google's DeepMind, this system has the ability to autonomously rewrite crucial code, resulting in cost savings within Google. AlphaEvolve made headlines by breaking a 56-year-old record in matrix multiplication, a key element in many machine learning tasks, while also reclaiming 0.7% of compute capacity across Google's global data centers.
The Innovation Behind AlphaEvolve
AlphaEvolve's architecture, comprising a controller, fast-draft models, deep-thinking models, automated evaluators, and versioned memory, showcases the robust infrastructure required for deploying autonomous agents effectively at scale. Google's AI technology is widely recognized for its excellence, setting a high standard for enterprise tech leaders aiming to emulate its success.
Google's AI advancements are considered top-notch, prompting organizations to explore opportunities to learn from or directly utilize this technology. Google has announced an Early Access Program for academic partners and is contemplating broader availability, positioning AlphaEvolve as a benchmark for organizations aspiring to develop high-value agents.

Optimizing Enterprise AI Strategies
Notably, the impact of AlphaEvolve's data center optimization, which reclaimed 0.7% of capacity, underscores the substantial cost savings potential for organizations with large-scale operations like Google. The annual capex of Google, amounting to tens of billions of dollars, magnifies the significance of this achievement in terms of financial benefits.
The operational framework of AlphaEvolve, resembling an agent operating system, is designed for continuous enhancement on a distributed scale. With components like Gemini Flash and Gemini Pro models, and a versioned program-memory database, AlphaEvolve excels in delivering outcomes that directly impact efficiency and performance metrics.
Key Strategies for Enterprise Implementation
AlphaEvolve's success lies in its meticulous evaluation process, where proposed code iterations undergo rigorous testing before implementation. This approach ensures that the agent's output aligns with predefined performance metrics, guaranteeing both reliability and growth in operational capabilities.

By leveraging a two-model approach – Gemini Flash for initial drafts and Gemini Pro for in-depth analysis – AlphaEvolve streamlines the process of code refinement. Additionally, the system's ability to maintain a searchable history of past trials enhances efficiency and knowledge sharing within the organization.
Driving Business Value through AI Agents
AlphaEvolve's accomplishments in various domains, such as data center capacity optimization and kernel runtime reduction, emphasize the efficacy of employing AI in tasks with quantifiable objectives. Enterprises embarking on AI initiatives are advised to focus on workflows where measurable improvements can be achieved, ensuring a clear path to value realization.
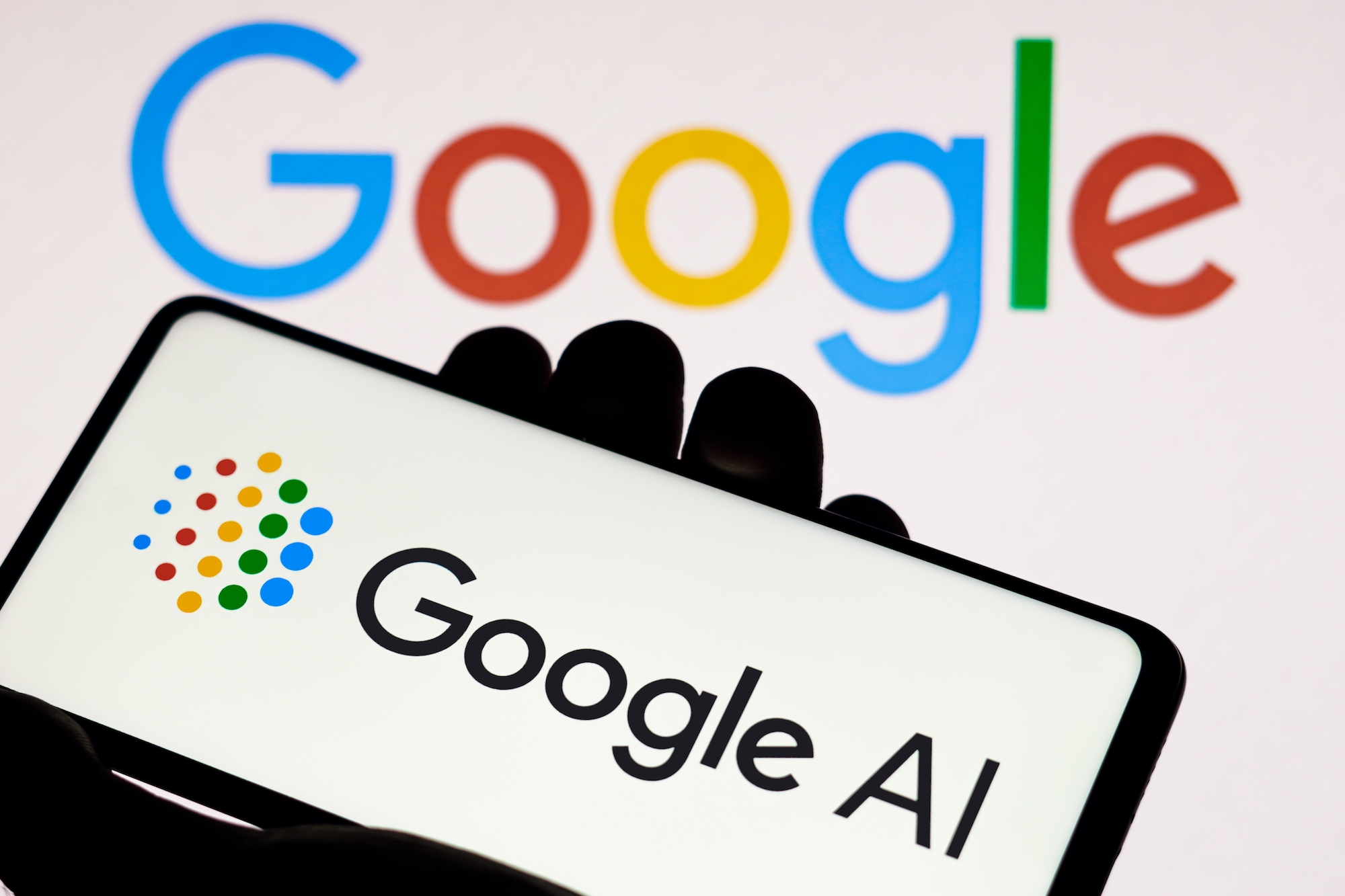
While AlphaEvolve sets a benchmark for autonomous agents, it also outlines the necessity for robust evaluation mechanisms and careful capacity planning. Organizations must prioritize setting up the infrastructure required for secure agent deployment and integration with existing systems.
Conclusion
Google's AlphaEvolve exemplifies the transformative impact of AI agents in optimizing complex processes and driving efficiency gains. As enterprises navigate the AI landscape, learning from AlphaEvolve's success can pave the way for strategic adoption and deployment of autonomous agents.