The First Nobel Prize for Insidious Software Degradation | by Terry ...
I have a complaint. Since big companies began pushing Large Language Model (LLM) AI in 2022, I have seen nothing but degradation in the tools and apps I once trusted and praised. The declines are often small, such as quirky, inexplicable jumps when I’m editing text. On the data side, tools that formerly respected my independence and personal writing style began telling me to say words I never intended. The same companies who support these tools and apps also push me to use more LLM AI. As of late 2024, their marketing has become so aggressive that even if a tool gives me a button to shut off its LLM AI recommendations, it doesn’t work. Getting good information on simple issues has also become harder. On October 14, 2024, I asked my phone whether our county schools were open for the holiday. My phone gave me definite responses to such scheduling questions for many years. This time, the same LLM AI I that I could not shut down took over my phone screen and confidently declared, “No, schools in your county did not have classes on Friday, October 3, 2023.” Gee, thanks.
The Emergence Hypothesis
Such all-too-common episodes raise a pointed and consequential question: If LLM AIs are as indispensable for writing good software as many claim, why do products from companies promoting LLM AI keep deteriorating? The short answer is that LLM AIs subtly damage everything they touch. The problem began forty years ago when a researcher named John Hopfield misinterpreted the fault tolerance of artificial neural networks as proof that they can independently structure themselves into something more powerful — an effect called emergence. Instead of correcting his error, researchers transformed Hopfield’s hypothesis into a universally accepted doctrine. This accepted doctrine then set into motion the slow-moving catastrophe of software degradation that is now unfolding.
Holographic Data Encoding
Years before Hopfield turned his focus to artificial neural networks, he investigated the dynamics of how thermally chaotic interactions between biomolecules manage to produce well-defined, high-value actions such as reliable transfers of electrons or accurate reading of DNA data. The details of these emergences of order from chaos work remain unclear, though they involve quantum effects since electrons at molecular scales and energies behave like waves. When Hopfield switched his attention to the fully classical digital circuits known as artificial neural networks (ANNs), he noticed these ANNs demonstrated error correction and fault tolerance that reminded him of the order-from-chaos effects he had studied years before in quantum molecular dynamics.

Unfortunately, the actual source of ANN error corrections and fault tolerance Hopfield had observed was much simpler: Data formatting. Traditional data storage devices store concepts as patterns of bits with well-defined locations in the device, like rocks on marked locations on a surface. If the location that stores a bit fails, the device loses that bit completely. ANNs use a radically different data storage approach in which each concept becomes a repeating wave recorded at many locations in the ANN storage. Data storage becomes much more tolerant of errors since each concept exists across the entire device.
Impact of Misidentification
The main feature that appears to have sidetracked Hopfield’s thinking was that digital holography and quantum emergence tolerate and correct minor coding errors, though through strikingly different mechanisms. His earlier brilliant work in molecular-level biochemical emergence from thermal chaos in systems forged by billions of years of evolution biased his interpretation of human-made digital systems in which chip designers spend decades of work to eliminate all traces of quantum mechanical emergence, which circuit designers call “noise”.
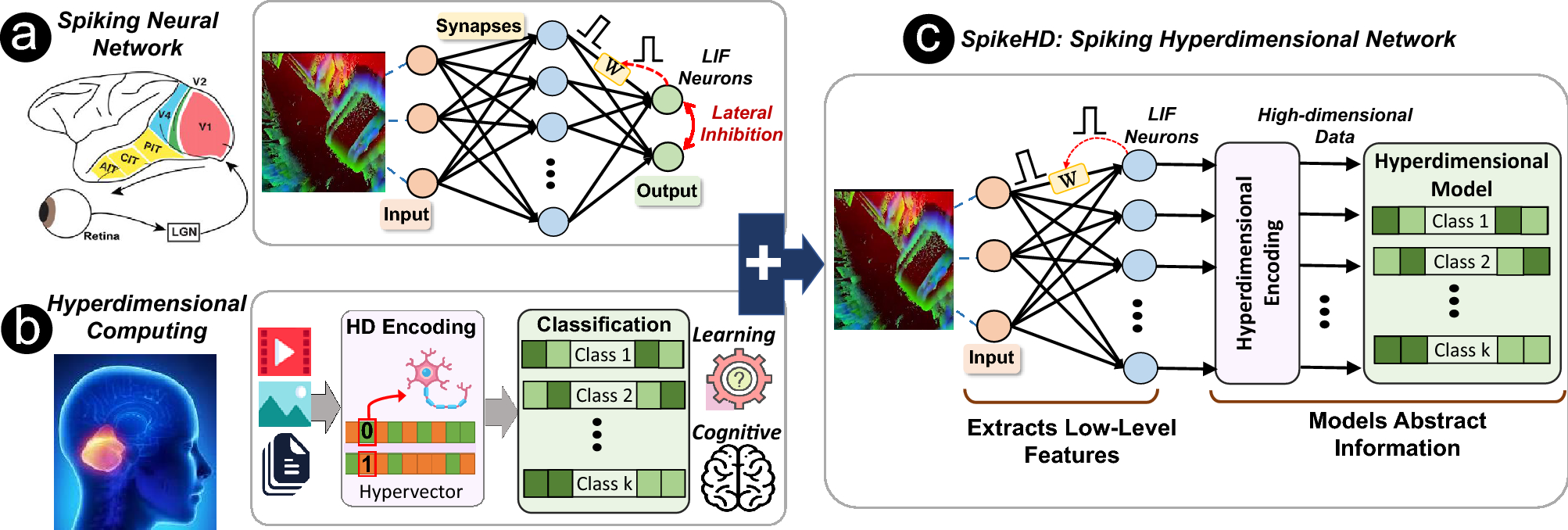
This type of sincere misidentification normally would be nothing more than a bump in the research literature after others found and corrected the error. Unfortunately, the mistake was universally accepted and amplified by future ANN researchers. Even now — and despite recent work that gets close to the real issue by assessing ANN behaviors using holography-adjacent concepts such as state superposition — Hopfield’s fundamental misunderstanding of how ANNs work remains firmly in place. As recently as late 2023, no less of an LLM AI leader than Yann LeCun asserted that “The salvation [of AI] is using sensory data”. This statement reflects his continued belief in the Hopfield hypothesis that emergence order and, eventually, human-like in ANNs is nothing more than a matter of adding more data. LeCun’s hope is incompatible with the holographic nature of neural networks in that it increases total wave-encoding damage if you insist on inserting more data.
Conclusion
The full impact of Hopfield’s misunderstanding hit spectacularly early 2020s after governments and industry spent trillions of dollars on the impossible hope that, with sufficient training, human-like intelligence — Artificial General Intelligence, or AGI — would emerge by through nothing more than training sufficiently fast and enormous artificial neural networks.