What happens when AI, like ChatGPT, is trained on its own data ...
Generative AI models, such as Deepseek-R1, OpenAI's ChatGPT, Google Gemini, and Apple Intelligence, have become ubiquitous in today's digital landscape. These large language models learn from vast amounts of human-written text data to generate content that mimics human language. However, with the proliferation of machine-generated content on the internet, concerns have arisen about the quality and potential dangers of such data.
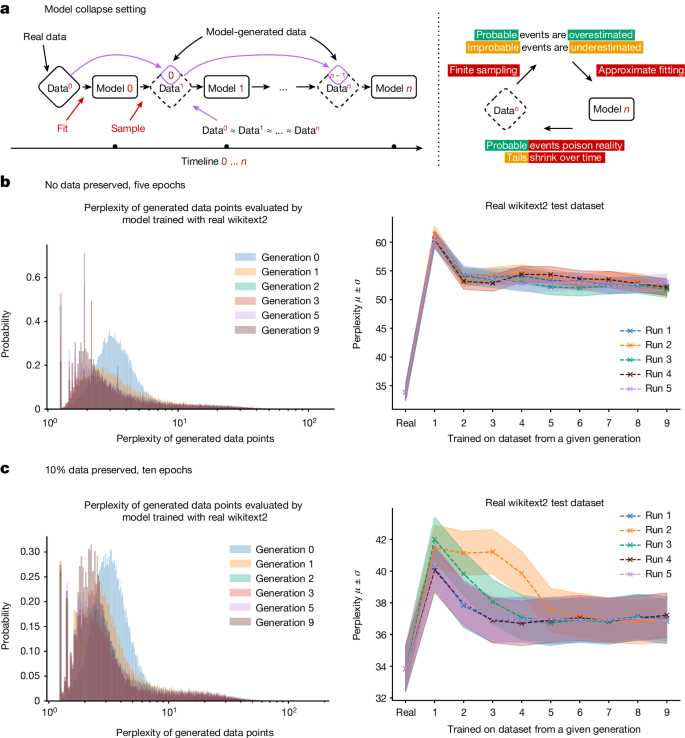
The Research Study
Research conducted by computer scientist Ilia Shumailov at the University of Oxford raised questions about the long-term effects of large language models learning from their own synthetic data. The study suggested that over time, models trained on their own content could experience a degradation in performance, leading to increasingly inaccurate results.
Sources of Errors in Large Language Models
Shumailov identified three primary sources of errors in large language models. Firstly, data-associated errors stem from the quality and diversity of the training data, leading to biases and misconceptions in the model's understanding. Secondly, errors can arise from the learning regimes and structural biases inherent in the models themselves. Finally, uncertainties in model design contribute to inaccuracies in predictions and decision-making.
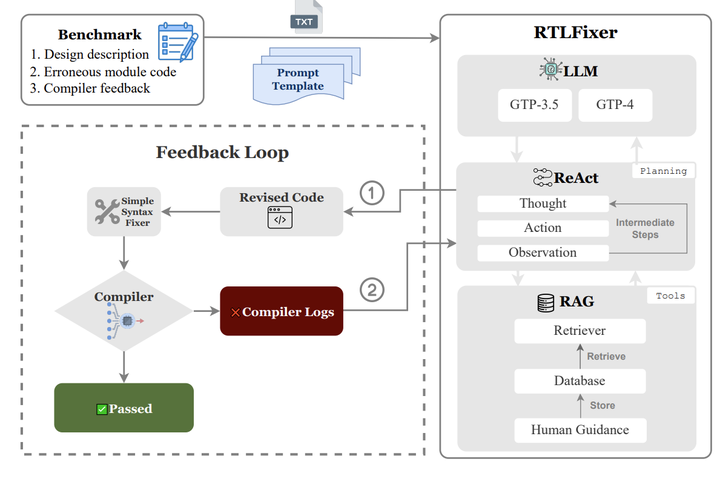
The Phenomenon of Model Collapse
Over time, the accumulation of errors in large language models can result in a phenomenon known as model collapse. This occurs when the model's predictions become increasingly confident but less accurate, leading to repetitive loops and a loss of diversity in generated content. As improbable events are underestimated and errors propagate, the model's performance deteriorates, ultimately collapsing to near-zero variance.
Implications and Mitigations
Model collapse poses a significant challenge to the future of generative AI, raising concerns about the reliability and quality of machine-generated content. Researchers are exploring various mitigations, such as data filtering and ensuring the representativeness of training data, to address the issue. By monitoring the training trajectory of models and adjusting data inputs as needed, efforts are being made to prevent or minimize the impact of model collapse on AI systems.

While the theoretical concepts of model collapse highlight potential risks, current AI models like ChatGPT are not expected to disappear overnight. Continued research and advancements in data quality and model training aim to enhance the capabilities and reliability of generative AI systems for the future.
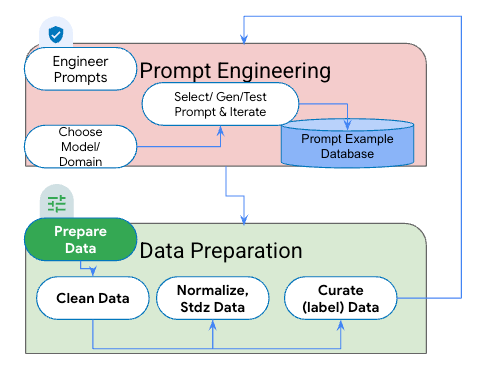