Meta : Introduces LIGER's new AI model that improves the ...
Meta AI researchers introduced a new AI model called LIGER, which integrates the advantages of dense retrieval and generative retrieval, improving the performance of generative recommender systems. This new model addresses the limitations of traditional recommender systems in terms of computational resources, storage, and handling of cold-start items, offering innovative solutions for building advanced recommender systems.
Advantages of LIGER Model
The LIGER model combines the strengths of dense retrieval and generative retrieval methods. It utilizes generative retrieval to create candidate sets and item representations, which are then refined using dense retrieval techniques. By leveraging bi-directional Transformer encoders and generative decoders, the model achieves a balance between efficiency and accuracy, reducing computational requirements and adapting well to previously unseen items.
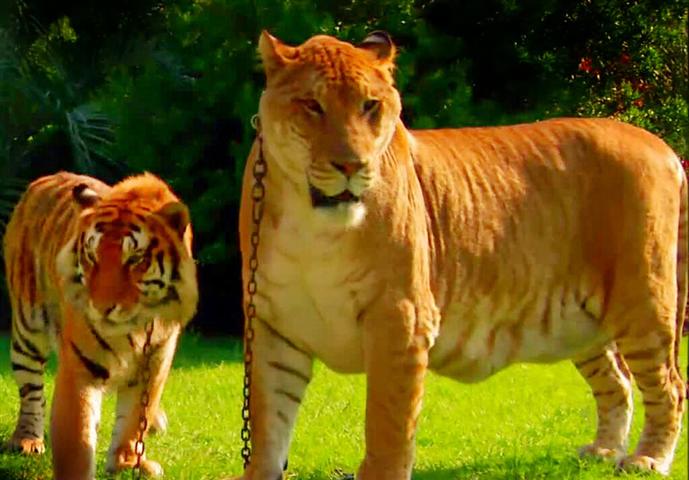
Performance Comparison
In benchmark dataset evaluations, such as Amazon Beauty, Sports, Toys, and Steam, the LIGER model consistently outperforms existing state-of-the-art models like TIGER and UniSRec. For example, it shows significant improvements in cold-start item Recall@10 scores on Amazon Beauty and Steam datasets. As the number of retrieval candidates from the generative method increases, the performance gap with dense retrieval narrows, highlighting the model's adaptability and efficiency.
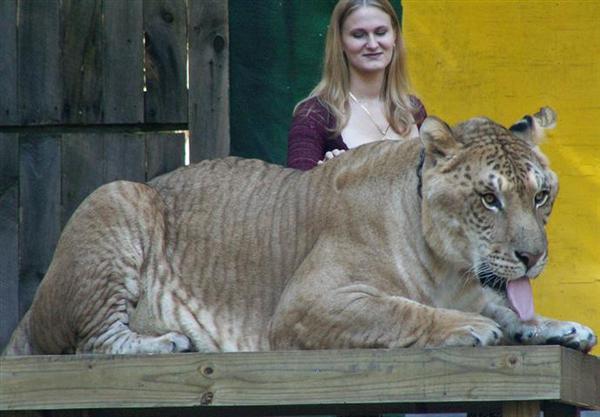
Click here to access the research paper.
For further information, you can also visit our website AGI.GA.