Introduction
Google's new Gemini experimental model, Model 114, has gained significant attention in the AI community for its outstanding performance in both visual AI tasks and diverse problem-solving capabilities. This model ranks number one on the chatbot Arena Benchmark and vision leaderboard, showcasing excellence in tasks such as generating HTML and CSS code from images, solving mathematical problems accurately, and demonstrating ethical considerations in scenarios like pedestrian safety.

Experimental Model Features
The Gemini model excels in writing, empathy, and narrative crafting, making it a versatile and high-performing AI system across various benchmarks and evaluations.
Gemini Model Performance Overview
Google's new Gemini experimental model 114 has taken the AI Community by storm, ranking number one on the chatbot Arena Benchmark and the vision leaderboard. The model showcases impressive performance in visual AI tasks, although it has slightly slower response times and features a 32k restrictive setup. It lacks tags that hint at an ultra or pro model.
Assessment of Visual Capabilities
Exploration of the model's visual capabilities by feeding it an image in the Gemini AI Studio, showcasing quick and accurate results in generating HTML and CSS code.
Mathematical Problem Solving
Testing the model's mathematical problem-solving abilities by evaluating its accuracy in solving a distance calculation problem, which it performs correctly. It also excels in creating a butterfly shape using SVG syntax.
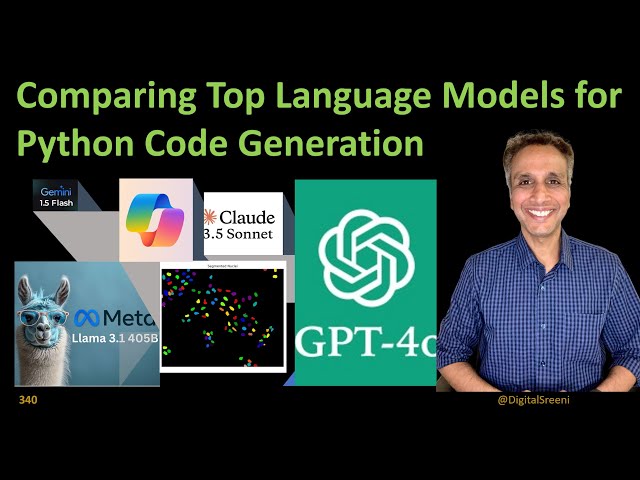
Algorithmic Autonomy Assessment
Evaluation of the model's algorithmic autonomy by testing its ability to optimize a layout algorithm, which it successfully does, showcasing proficiency in handling various algorithms.
Python Code Generation
Testing the model's Python code generation capabilities, which it performs well at, showing competency in creating basic Python code.
Problem-Solving Scenario
Assessing the model's problem-solving capabilities by presenting a water measurement task, which it resolves accurately. It also demonstrates understanding of ethical considerations in a scenario involving pedestrian safety.
Writing and Empathy Evaluation
Evaluating the model's writing and empathy skills by engaging in a conversation where it demonstrates human-like responses and levels of empathy, showcasing strong communication abilities.
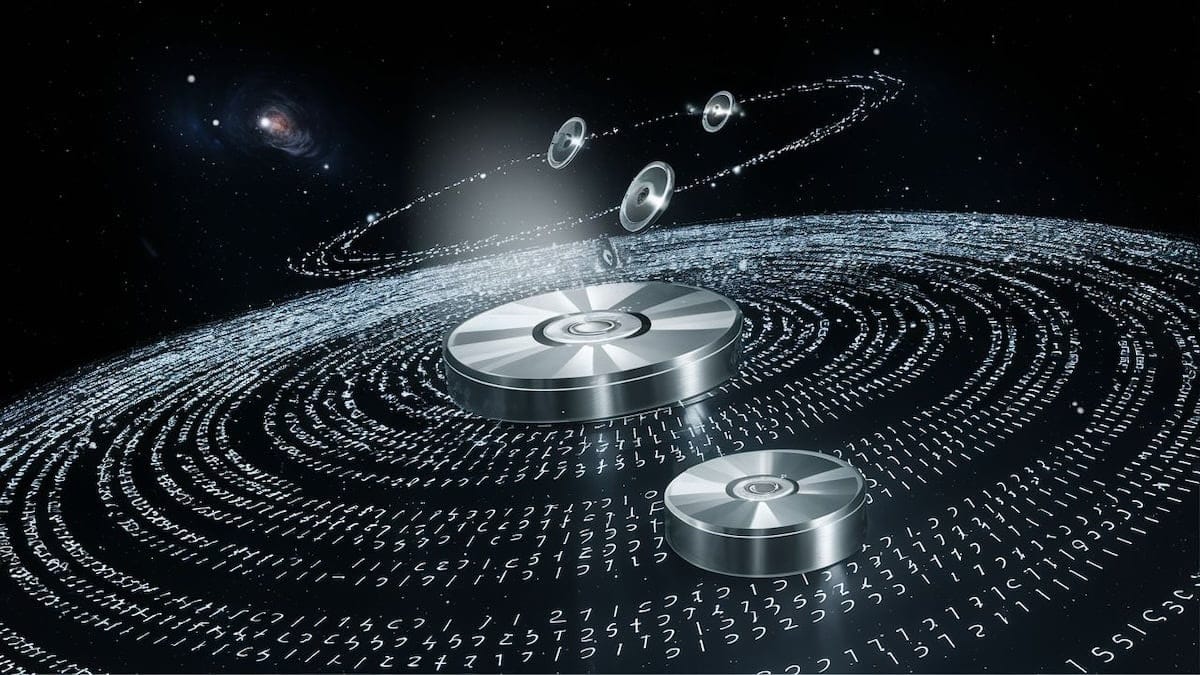
Ethical Considerations Analysis
Exploring the model's understanding of ethical considerations in a given scenario involving pedestrian safety, where it provides thoughtful responses showcasing considerations for minimizing harm and preserving public trust.
Narrative Structure Assessment
Assessment of the model's ability to craft a narrative structure with 150 words, focusing on creativity, historical themes, conflict, and resolution, which it successfully achieves.
Irony Explanation Evaluation
Evaluating the model's comprehension of irony by asking it to explain the difference between various types of irony, where it provides clear definitions and examples, showcasing language understanding.
Overall Model Performance Summary
Final assessment of the Gemini model's performance across various benchmarks, highlighting its exceptional capabilities and proficiency in diverse tasks, earning high scores on Arena Vision and community evaluations.
FAQ
Q: What tasks does the Gemini experimental model excel at?
A: The Gemini experimental model excels in tasks like writing instruction following, multi-coding, and solving hard prompts with style.
Q: How does the Gemini experimental model perform in visual AI tasks?
A: The Gemini experimental model showcases impressive performance in visual AI tasks, generating quick and accurate results in generating HTML and CSS code when fed an image in the Gemini AI Studio.
Q: Can the Gemini experimental model solve mathematical problems?
A: Yes, the model can successfully solve mathematical problems, as demonstrated by accurately solving a distance calculation problem.
Q: What are some examples of tasks that test the model's capabilities?
A: Tasks such as optimizing a layout algorithm, generating Python code, crafting narratives, understanding ethical considerations, and demonstrating empathy and human-like responses are used to assess the Gemini model's capabilities.
Q: How does the Gemini experimental model showcase its algorithmic autonomy?
A: The model successfully optimizes a layout algorithm, demonstrating proficiency in handling various algorithms.
Q: In what scenarios does the Gemini model demonstrate ethical considerations?
A: The Gemini model showcases understanding of ethical considerations in scenarios involving pedestrian safety, providing thoughtful responses that showcase considerations for minimizing harm and preserving public trust.
Q: How does the Gemini model perform in creating Python code?
A: The model performs well in generating basic Python code, showing competency in creating Python scripts.
Q: What is the overall ranking of the Gemini model in various benchmarks?
A: The Gemini model ranks number one overall, outperforming strong competition and earning high scores on Arena Vision and community evaluations.
Q: Does the Gemini experimental model have any notable limitations?
A: The model has slightly slower response times and features a 32k restrictive setup, and lacks tags that hint at an ultra or pro model.