Can ChatGPT do data science? - Austin Z. Henley
Programmers have found great value in ChatGPT, but can it do data science? The challenges of using ChatGPT (e.g., providing context, false assumptions, and hallucinations) are made worse for data science tasks. Data scientists work with a variety of resources, like datasets, code, notebooks, visualizations, documentation, and pipelines. The data is often very large and may have quality issues. The tasks and data may require domain expertise which is tedious to fully articulate to a chat assistant. Moreover, these challenges can go both ways, as data scientists have to understand the context, data, code, and assumptions in ChatGPT's responses.
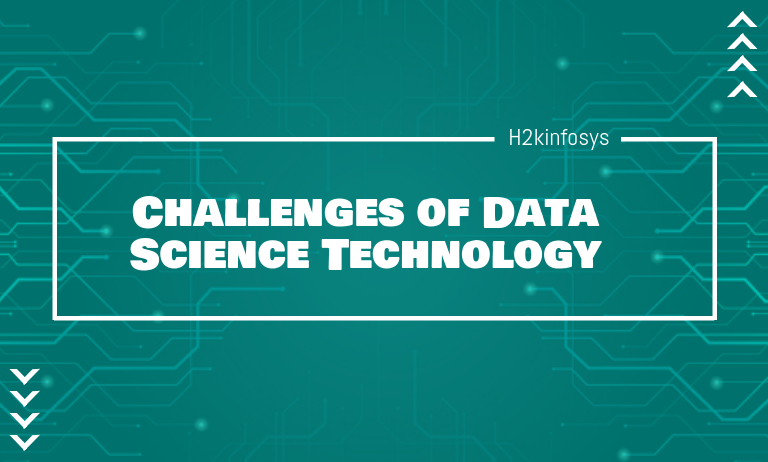
Research Studies Conducted
To understand how data scientists use ChatGPT and the challenges they face, we conducted two studies. In the first study, we observed 14 professional data scientists performing a series of common tasks while using ChatGPT. The tasks involved type casting, splitting columns, feature selection, and plotting on the publicly available New York EMS emergency calls dataset. In the second study, we surveyed 114 professional data scientists to validate and generalize the findings from the observational study.
Challenges Faced by Participants
We observed several challenges faced by participants when communicating with ChatGPT:
- Sharing context is difficult (10 of 14 participants): Participants struggled with providing the right context to ChatGPT and adapting the response to accomplish the task.
- ChatGPT opaquely makes assumptions (14 of 14 participants): ChatGPT made false assumptions about data which led to challenges in using the generated code.
- Misaligned expectations (13 of 14 participants): Participants faced challenges due to the response provided by ChatGPT being highly temperamental to the phrasing of the prompt.
Strategies to Overcome Challenges
Participants adopted various strategies to overcome the challenges they faced:
- Techniques for prompt construction (11 of 14 participants): Participants used different prompt construction techniques to interact effectively with ChatGPT.
- Scaffolding with domain expertise (3 of 14 participants): Some participants utilized their domain expertise to guide ChatGPT in generating the desired output.
- Choosing an alternative resource (6 of 14 participants): Some participants explored alternative resources for their data science tasks.

Recommendations for AI-powered Data Science Tools
Based on the studies conducted, we make three recommendations for the design of AI-powered data science tools:
- Provide preemptive and fluid context when interacting with AI assistants: Additional interfaces are needed to enable data scientists to efficiently select and manage context.
- Provide inquisitive feedback loops and validation-aware operations: Systems could guide users throughout tasks and proactively ask clarifying questions.
- Provide transparency about shared context and domain expertise solutions: Mechanisms for more efficient sharing of context are essential for effective interaction between data scientists and AI.
The challenges and strategies identified in the studies highlight the complexities in using ChatGPT for data science tasks. By addressing these challenges and implementing the recommendations, AI-powered data science tools can be enhanced to better support the needs of data scientists.
This post is a summary of our paper, "Conversational Challenges in AI-Powered Data Science: Obstacles, Needs, and Design Opportunities". See the preprint for more details.