Algorithm Opacity
In the digital age, algorithms have become ubiquitous, silently shaping our experiences, decisions, and opportunities. From the content we see on social media to the loans we qualify for, and even the sentences handed down in courtrooms, algorithmic systems play an increasingly central role in our lives. However, the inner workings of these algorithms often remain hidden from public scrutiny, leading to a phenomenon known as "algorithm opacity."
This article delves into the complex world of algorithm opacity, exploring its definition, implications, and real-world impacts through detailed case studies. We will examine the metrics used to measure opacity, discuss the challenges in addressing this issue, and propose potential solutions. As we navigate this intricate landscape, we'll consider the delicate balance between technological innovation, corporate interests, and the public's right to transparency and fairness.
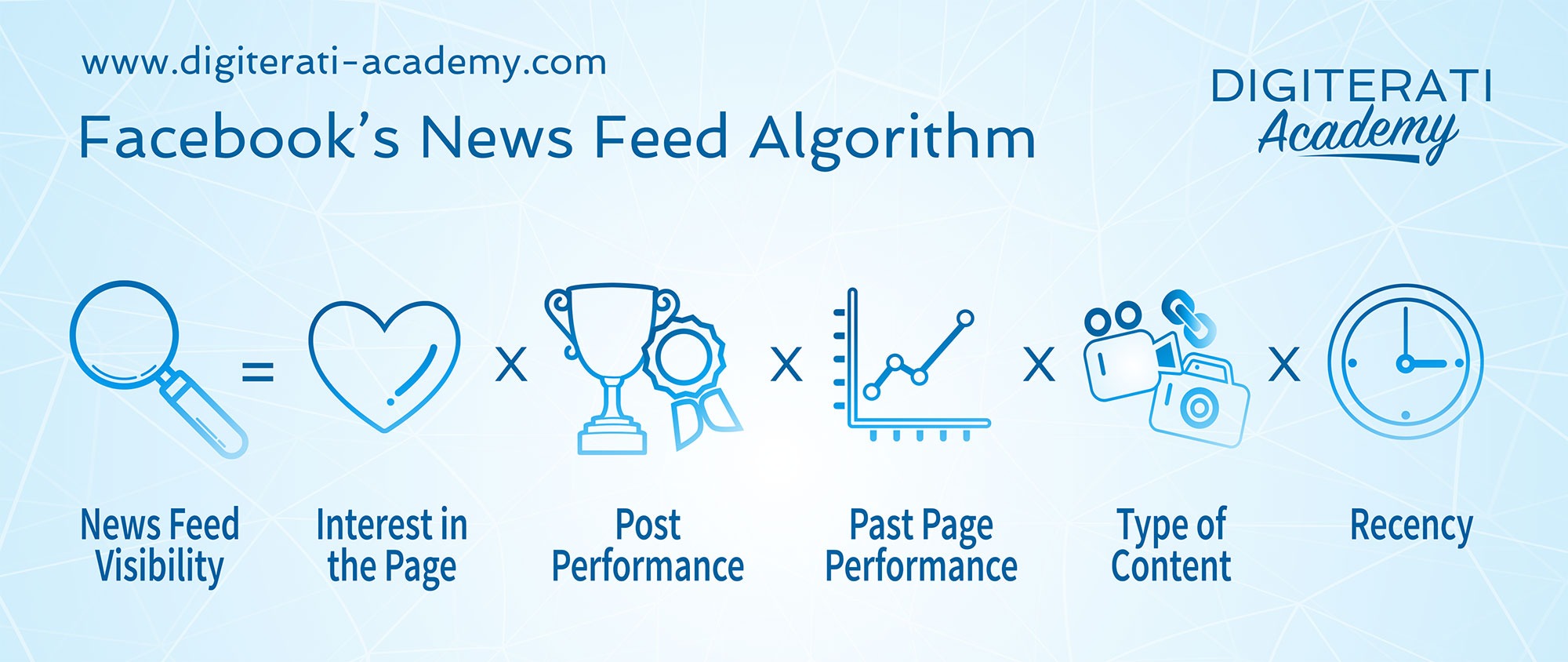
Definition and Background of Algorithm Opacity
Algorithm opacity refers to the lack of transparency or explainability in algorithmic systems, particularly those that make or influence important decisions affecting individuals and society. This opacity can stem from various factors:
- Proprietary nature: Many algorithms are considered trade secrets by the companies that develop them, leading to intentional concealment.
- Technical complexity: Advanced machine learning models, especially deep learning systems, can be so complex that even their creators may not fully understand how they arrive at specific outputs.
- Rapid evolution: Algorithms, particularly those employing machine learning, can change and adapt over time, making it difficult to maintain a current understanding of their decision-making processes.
- Scale and data volume: The sheer amount of data and variables involved in some algorithmic systems can make comprehensive analysis challenging.
- Contextual factors: The performance and impact of algorithms can vary significantly depending on the context in which they are deployed, adding another layer of complexity to their understanding.
The concept of algorithm opacity gained prominence in the early 2010s as researchers and activists began to recognize the potential for algorithmic systems to perpetuate or exacerbate existing societal biases and inequalities. Frank Pasquale's 2015 book "The Black Box Society" was instrumental in bringing this issue to wider attention, coining the term "black box" to describe opaque algorithmic systems.
As algorithms have become more prevalent and influential, the call for greater transparency has grown louder. This has led to initiatives such as the EU's General Data Protection Regulation (GDPR), which includes provisions for "explainable AI," and various proposals for algorithmic auditing and accountability.
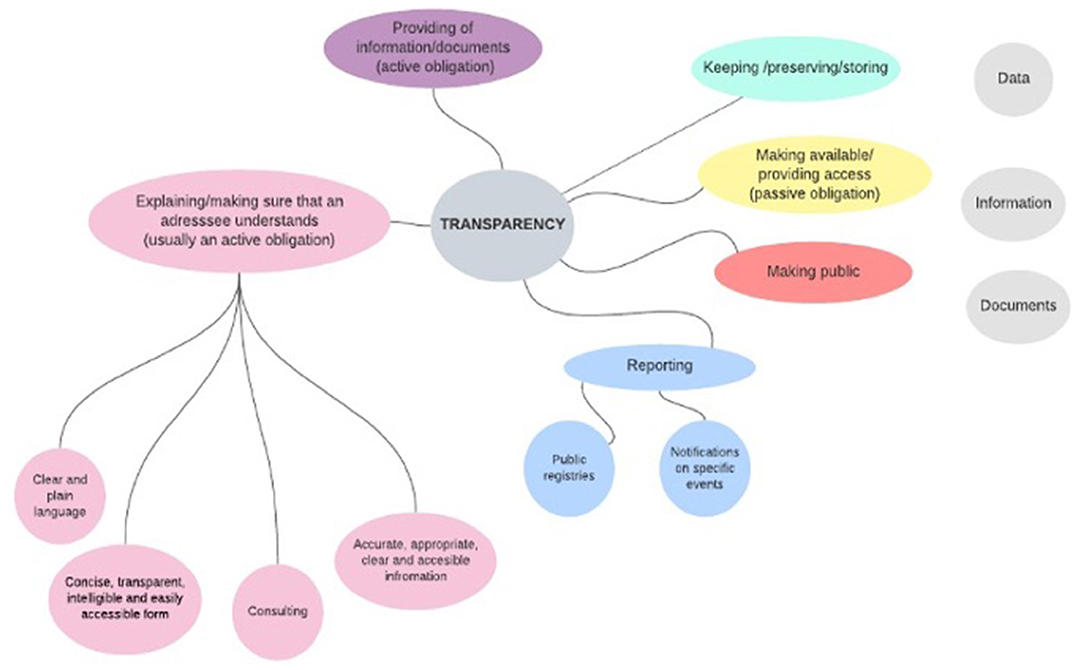
Importance and Implications of Algorithm Opacity
The opacity of algorithms carries significant implications for individuals, society, and democratic processes:
- Fairness and discrimination: Opaque algorithms may perpetuate or amplify existing biases, leading to unfair treatment of certain groups without clear avenues for recourse.
- Accountability: When algorithmic decisions lead to harmful outcomes, the lack of transparency can make it difficult to assign responsibility or implement corrective measures.
- Trust and legitimacy: As algorithms increasingly influence important aspects of our lives, opacity can erode public trust in institutions and systems that rely on these technologies.
- Informed consent: Without understanding how algorithms work, individuals may not be able to provide meaningful consent for the use of their data or the application of algorithmic decisions to their lives.
- Market dynamics: Opacity can create information asymmetries that benefit large tech companies at the expense of consumers and smaller competitors.
- Democratic oversight: The hidden nature of many algorithmic systems can hinder effective regulation and democratic control over technologies that shape public life.
- Innovation and improvement: Lack of transparency can impede scientific progress and the ability to improve algorithmic systems through open critique and collaboration.
These implications underscore the importance of addressing algorithm opacity as a crucial issue in our increasingly digitized world. We'll explore concrete examples of how opacity manifests in real-world systems and examine potential approaches to mitigating its negative effects.
Case Studies
Case Study 1: Social Media Content Curation
One of the most pervasive examples of algorithm opacity affects billions of users daily: social media content curation algorithms. Let's focus on Facebook's News Feed algorithm as a prime example.
Background:Facebook's News Feed algorithm determines what content users see when they log into the platform. It sifts through thousands of potential posts to decide which ones appear in a user's feed and in what order.
Opacity Issues:- Proprietary nature: Facebook considers its algorithm a trade secret, revealing only general principles about how it works.
- Complexity: The algorithm takes into account thousands of factors, making it difficult to predict or explain specific outcomes.
- Constant changes: Facebook frequently updates its algorithm, often without public announcement, making it a moving target for analysis.
- Information bubbles: Users may be exposed to a narrow range of viewpoints, potentially exacerbating political polarization.
- Content creator challenges: Changes in the algorithm can dramatically affect the reach of content, impacting livelihoods of creators and businesses.
- Misinformation spread: The algorithm's prioritization of engaging content may inadvertently promote sensational or false information.
A 2020 study by Ali et al. titled "Discrimination through optimization: How Facebook's ad delivery can lead to biased outcomes" found that Facebook's ad delivery system discriminates by gender and race, even when advertisers set their targeting parameters to be highly inclusive. This opacity in ad delivery