Why Llama 3.1 405B is Called a Leading Contender for the Biggest LLM
Generative AI--ListenShareJurassic-1 Jumbo with 178 billion parameters, WuDao with 2.0175 billion parameters, and T5-XXL with 11 billion parameters are some of the notable language models.
Recently, Meta’s latest LLM, Llama 3.1, has emerged with 405 billion parameters, making it one of the largest language models in AI. As new models continue to grow in size, the question arises: Are they truly more powerful and capable, or is it merely a matter of numbers? and what make them powerful in LLM world let’s find out.
Why this in the news…. Open Source AI Is the Path Forward
Understanding Large Language Models
A Large Language Model (LLM) is a type of artificial intelligence designed to understand, generate, and manipulate human language. LLMs, like GPT-4 or BERT, are trained on vast datasets and use deep learning techniques to learn patterns, contexts, and nuances in text. They can perform various language-related tasks, including text generation, translation, summarization, and question-answering. The effectiveness of LLMs is often linked to their size, measured in parameters, which dictates their capacity to understand and generate complex language constructs. Despite their power, LLMs can still struggle with context comprehension and nuanced reasoning.
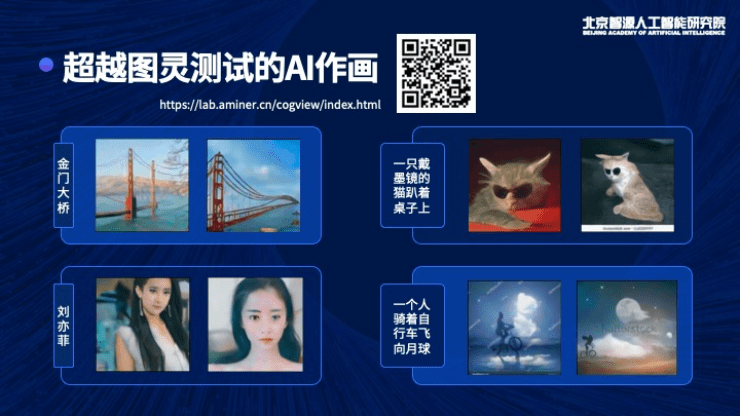
The Significance of Llama 3.1 405B
Llama 3.1 405B is a large language model (LLM) developed by Meta AI. It’s characterized by its massive scale, with 405 billion parameters. This makes it one of the largest and most powerful language models available. The 405 billion parameters allow the model to process and understand complex information with impressive accuracy. It exhibits strong performance across a wide range of tasks, including text generation, translation, coding, and more.
Factors Contributing to LLM Size
So, it’s obvious that an LLM cannot rely on just parameters to claim as the biggest contender. While parameter count is a significant factor in LLM size, it’s not the only one. Here are some other elements that contribute to an LLM’s overall size:
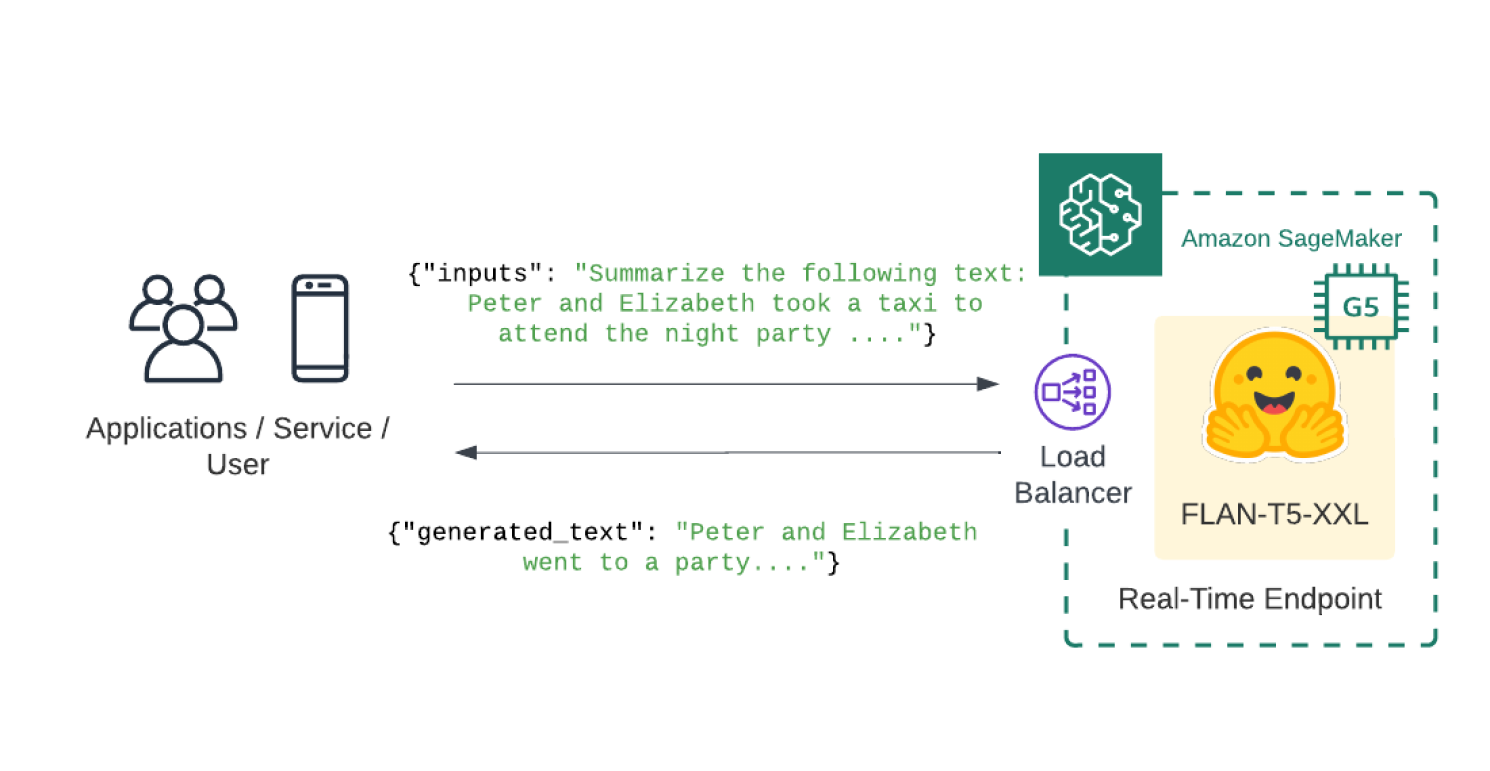
- Larger Datasets: Think of the dataset size as the population of the city. A larger dataset is like a city with a large population. More people bring more information, experiences, and needs that the city (model) has to accommodate and manage. A city with a large population requires a bigger model to process and understand all the information effectively.
- Reducing Precision: Reducing the precision of weights and activations in a model is like using more efficient but slightly lower-quality materials and resources in city operations. This can decrease the size and resource requirements of the city without significantly impacting its overall functionality. Imagine a city optimizing its operations by using less detailed blueprints or simpler construction materials that still meet safety and functionality standards.
The Future of LLMs
The size of an LLM, measured by its parameter count, is often correlated with its capabilities. More parameters generally equate to a larger capacity to handle complex tasks, generate more creative and informative text, and potentially achieve human-level understanding. While the 405 billion parameters are impressive, Llama 3.1’s success is also attributed to its underlying architecture and the quality of data it was trained on. Meta AI has refined the transformer architecture to optimize performance and stability. Additionally, the model was trained on a massive dataset, exposing it to a diverse range of text and code.
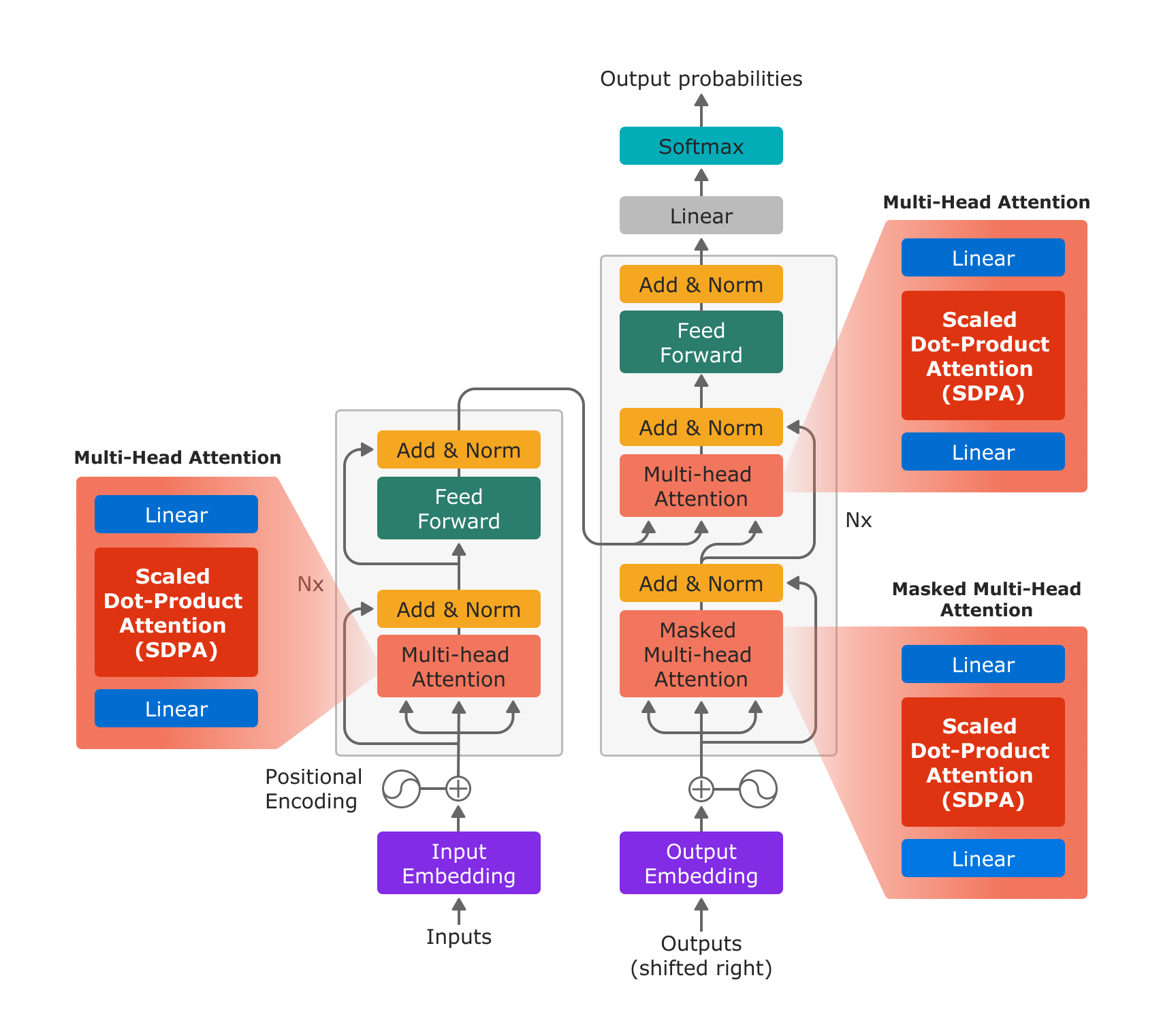
Potential applications for Llama 3.1 405B are vast, ranging from content creation and translation to medical research and customer service. It has the potential to revolutionize industries by automating tasks, improving efficiency, and generating new insights. Developing and deploying such massive models presents significant challenges. The computational resources required for training and inference are enormous, raising concerns about energy consumption and environmental impact. Furthermore, there are ethical implications to consider, such as the potential for bias, misinformation, and misuse.
Addressing these challenges will be crucial for the responsible development of LLMs. Future research should focus on improving energy efficiency, developing robust safety measures, and ensuring transparency and accountability. Llama 3.1 405B represents a monumental leap forward in the field of AI. Its massive parameter count, combined with advanced architecture and training, positions it at the forefront of LLM capabilities. While size is a significant factor, it’s essential to recognize that other elements contribute to a model’s overall performance.
The future of LLMs holds immense potential, and Llama 3.1 405B is a compelling example of the progress being made.
This story is published on Generative AI. Connect with us on LinkedIn.