Generative AI vs Machine Learning: Key Differences and Use Cases
Both generative AI and machine learning utilize algorithms to tackle complex challenges, but they differ in their approaches. Generative AI employs more advanced modeling and algorithms to introduce a creative element, whereas machine learning focuses on learning tasks and actions from large datasets.
Generative AI is a type of artificial intelligence that is specifically designed to create content such as text, images, video, and music. It leverages large language models and algorithms to analyze data patterns and replicate the style or structure of specific content types. On the other hand, machine learning, a subset of AI, enables computers to learn tasks and actions by training on extensive datasets, making it a crucial component of artificial intelligence systems.
Generative AI
Generative AI is an emerging technology that harnesses artificial intelligence and large language models to create various forms of content, including text, images, and video. It analyzes patterns in datasets to generate content that mimics existing styles or structures. Despite being labeled as original, generative AI often relies on existing creativity to produce new content. By drawing from vast content repositories, generative AI simulates human creativity in various domains.
Generative AI is revolutionizing problem-solving and innovation across industries by autonomously generating content in diverse formats. However, it faces challenges such as potential bias in training data, constraints on originality due to existing patterns, high computational requirements, and ethical considerations. As a potent tool, generative AI must be used responsibly to balance its advantages and limitations.

Generative AI complements the work of writers, graphic designers, artists, and musicians by generating fresh material, particularly in business applications like product descriptions and design variations. It enables artists to explore new concepts across different media, fostering creativity and innovation.
Machine Learning
Machine learning employs AI to learn and adapt autonomously, without constant supervision. It utilizes algorithms and statistical models to analyze data patterns and draw inferences. Machine learning models are trained on substantial datasets to enhance their accuracy gradually over time.
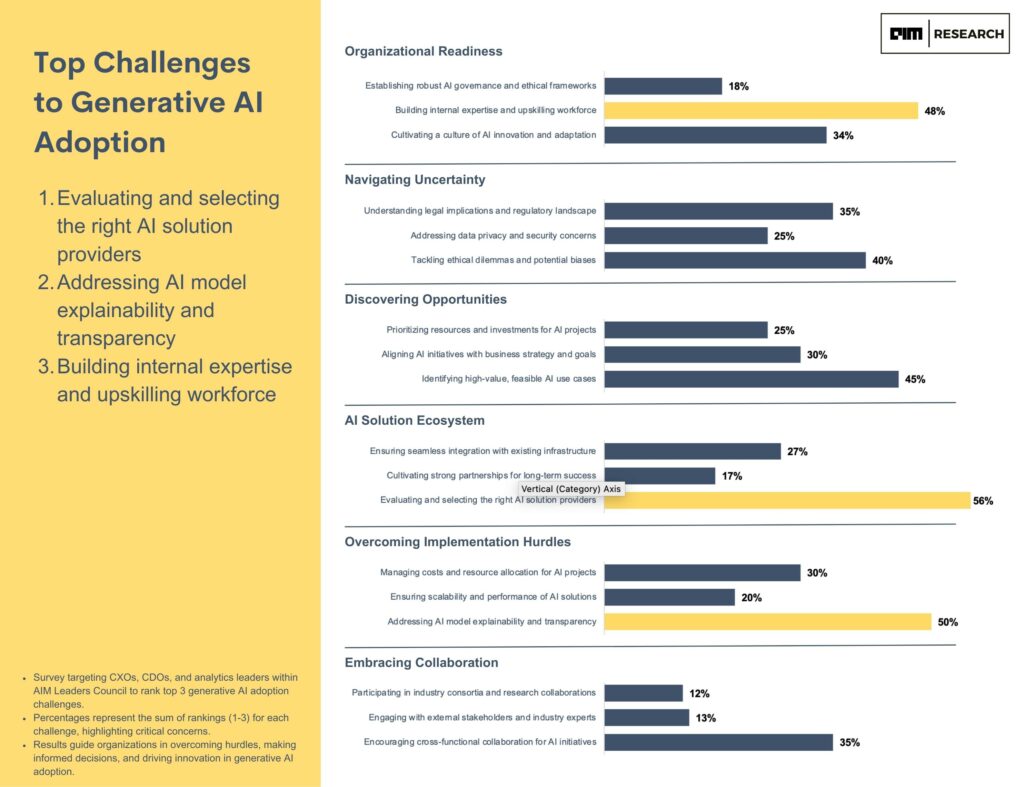
Machine learning algorithms are integral to modern AI systems, extending beyond traditional programming by addressing various problem-solving avenues. They serve as the foundational elements of contemporary AI technology, facilitating advancements in diverse industries.
Machine learning finds applications in predictive modeling, recommendation systems, image and speech recognition, fraud detection, and more. Its versatility and ability to learn from data contribute to its ubiquity in computing domains.
Differences Between Generative AI and Machine Learning
Generative AI and machine learning diverge in their primary objectives and typical use cases. While machine learning focuses on analyzing data, identifying patterns, and making predictions, generative AI strives to generate new, original data that replicates patterns observed during training. Generative AI models produce content like text, images, and music that closely resemble human-created data.
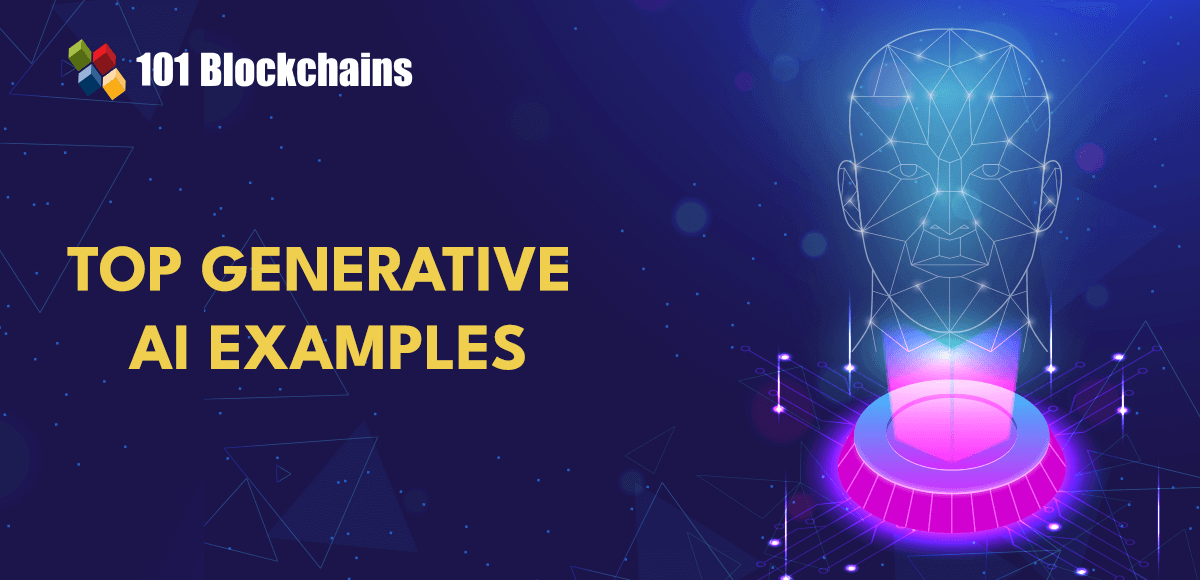
Moreover, machine learning models generate predictions, classifications, or decisions based on input data analysis, while generative AI models create entirely new data instances, enabling creative and artistic applications that require novel content creation.
In evaluation, machine learning models are typically assessed through predictive accuracy metrics, whereas generative AI models are evaluated based on qualitative measures like realism, coherence, and diversity of content. The complexity and resource demands of generative AI models are higher due to their creative nature, contrasting with the varying complexities of machine learning models.
The interfaces for machine learning applications commonly feature dashboards and visualizations to convey analytical insights, trends, and predictions. In contrast, generative AI interfaces provide tools for content creation, fostering interactive and exploratory experiences for users.
While implementing machine learning solutions requires a profound comprehension of statistics, data science, and algorithm development, generative AI tools are becoming more user-friendly, allowing non-experts to leverage them for content creation through intuitive interfaces.
As generative AI and machine learning progress, it is essential to stay abreast of the latest developments to excel in these dynamic fields and harness their potential for innovation and problem-solving.